A promising DNA methylation analysis pipeline for epigenetic studies and clinical implementation in inflammatory bowel disease
Highlight box
Key findings
• We proposed an improved DNA methylation (DNAm) analysis pipeline performing accurately on a public dataset regarding age prediction and cell type deconvolution, which could been seen by significant correlations with the ground truth (R2=0.96) and (highest R2=0.99) of phenotype of age and cell type deconvolution, respectively.
What is known and what is new?
• DNAm is the most studied epigenetic change and its correlation to inflammatory bowel disease (IBD) pathogenesis has been established. However, the newest DNAm analysis framework (MethyNet) were designed to deal with methylation data generated from microarray-based technologies only.
• In addition to reviewing the association between epigenetic mechanisms with IBD pathogenesis, we also have proposed a comprehensive DNAm analysis pipeline able to process data from all available platforms in the world.
What is the implication, and what should change now?
• Our methylation analysis pipeline could be used in processing DNAm data generated from different technologies to classify patients and healthy people. Thus, this could be potential to apply in IBD diagnosis tools using DNAm data.
• We expected to build up a IBD dataset to accelerate the process of developing a new diagnostic method utilizing our pipeline.
Introduction
Incidence, prevalence, and demographics of inflammatory bowel disease (IBD)
IBD which includes Crohn’s disease (CD) and ulcerative colitis (UC) poses great challenges to global healthcare with a soaring incidence. In 2018, the global incidence of IBD was 0.3%, and it is also predicted that the figure will be 0.9% in 2025 (1,2). The highest incidence rates were witnessed in Europe and North America (e.g., Canada, Denmark, Germany, Sweden, UK and USA). Simultaneously, although several studies have reported a lower incidence in Asia regions, 1.37 per 100,000 individuals in the general Asian population, these numbers have experienced a significant increase over a few past years (3). Besides, in Asia-Pacific, the median age at IBD diagnosis was 39 years (range, 5–81 years) for all patients, and males made up 57.6% of patients. Despite the unclear pathogenesis of IBD, it is well documented that genetic, environmental and host-related factors play critical roles in the development of both UC and CD (4). An on-going project is conducted in 29 regions globally with the aim to identify the incidence, demographic factors and environmental factors associated with IBD, especially in areas lacking epidemiology data including Vietnam (5).
Genetic factors of IBD have been studied for a considerable time with findings from genome-wide association studies indicating more than 201 genetic variations, including 41 CD-specific polymorphisms, 30 UC-specific polymorphisms, and 137 loci attributed to both CD and UC (6,7). However, these results could not completely explain the etiology, complexity and evolution of the disease. One of the reasons can be 80% to 90% of such identified loci are noncoding, indicating the vital role of the environmental components, especially epigenetic factors (8). Environmental factors including diet, infection, and medications all of which may lead to intestinal inflammation through their effects on the composition of the microbiome (8). A host-microbe interaction study on IBD has found the number of IBD-associated gene loci to 163, of which the predominance are associated with both phenotypes, 30 CD specific and 23 UC specific (6). Many studies reported the change of gut flora in both phenotypes compared to healthy controls as significantly reduced biodiversity, especially the decrease of Firmicutes and Bacteroidetes. In CD, the enterobacteria was over-represented while in UC, the reduction of Clostridium spp. and the increase of Escherichia coli (E.coli) was reported. Therefore, the understandings on the pathogenesis mechanisms and interactions among factors promise to optimize IBD treatment in multidisciplinary approaches (9,10).
Epigenetic mechanisms and their relationship with IBD
For a long time, researchers had believed that DNA sequences are the underlying factor determining the phenotypes of cells. However, they have found that despite the fact that somatic cells with the same origin share the entire genome, they still serve as completely distinct functions. The term “epigenetics” refers to a scientific field accounting for processes that influence gene activity without altering the sequence of DNA, enriching our understanding of the expression of various diseases (11). Epigenetic factors have been proven to be involved in the pathogenesis of a variety of disorders, such as cancers, cardiovascular diseases, and autoimmune diseases (12-14). The association between epigenetic modifications and disease susceptibility, severity and progression could suggest novel targets for treatment or potentially introduce more effective approaches for the disease diagnosis and management. The primary epigenetic processes regulating gene expression consist of DNA and RNA methylation, histone modifications, short and long non-coding RNAs. In the later part, we will introduce DNA methylation (DNAm) and demethylation, RNA methylation and other concepts in epigenetics and their association with IBD.
DNAm and demethylation
The most thoroughly investigated epigenetic mechanism is DNAm. DNAm is the covalent attachment of a methyl (CH3) group to pyrimidine ring of cytosine at the carbon 5 position, this modification preferentially occurs in 5'CG3' (CpG islands-CGI), some involves CpA and CpT as well (15). DNAm is performed by DNA methyltransferases (DNMT) family including DNMT1, DNMT1b, DNMT1o, DNMT1p, DNMT2, DNMT3A, DNMT3B and its isoforms as well as DNMT3L (16). De novo methylation (methyl groups are added to unmethylated DNA) are performed by DNMT3A and DNMT3B, while DNMT1 is responsible for maintenance methylation (when CpG dinucleotides on one strand are methylated) (17).
Aside from methylation, there is a reverse process called demethylation, which could recover the expression of silenced genes influenced by DNMT. The enzymes participating in this mechanism include 5-methylcytosine glycosylase, and an enzyme group of Ten-eleven translocation methylcytosine dioxygenases (e.g., TET1, TET2, and TET3), which removes the methylated cytosine from DNA and add back another cytosine in nucleotide form, or turn 5-methylcytosine (5mC) to 5-hydroxymethylcytosine (5-hmC), eventually to 5-formylcytosine (5-fC) and 5-carboxylcytosine (5-caC) (16). The balanced occurrence of methylation and demethylation serves as a dynamic mechanism controlling gene expression in many cell types (18).
Several studies have been conducted with a design of epigenome-wide association studies (EWAS) or focused on analysis of the methylation features of selected candidate genes to explore the pathogenesis of IBD by employing samples derived from peripheral blood and intestinal tissue of IBD patients (19-22). The first EWAS studying the changes in DNAm profile in IBD patients was reported by Nimmo et al. [2012] (21). They revealed a methylation profile of the whole genome from blood samples of 21 CD patients and 19 healthy controls, then reported several associated loci (including MAPK13, FASLG, PRF1, S100A13, RIPK3, and IL-21R).
Cooke et al. [2012] reported a EWAS for significant difference in methylation profile (THRAP2, FANCC, GBGT1, DOK2 and TNFSF4) of IBD patients compared to healthy controls and they also demonstrated that a majority of risk loci identified by GWAS (such as CARD9, CDH1, ICAM3, etc.) pose methylation changes between IBD patients and healthy individuals, indicating the potential for mechanistic interactions between genetic and epigenetic signals (22). This finding suggested that these single nucleotide polymorphisms (SNPs) may present in CGIs and influence the methylation statuses of CGIs. In addition, the changes in methylation patterns in the vicinity of susceptibility genes’ transcription initiation site and promoter region could have a significant impact on how genes are transcribed (23).
Recently, a few research teams have revealed diagnostic models for IBD based on methylation patterns. Ventham et al. [2016] generated a panel of 30 methylation probes that could distinguish IBD patients and healthy controls with a sensitivity of 75% and a specificity of 100% (24). Another methylation-based model developed by Howell et al. [2018] has shown the ability to differentiate CD patients from UC in 77% cases and area under the receiver operating characteristic curve (AUC) =0.92 (sensitivity =57%, specificity =100%) (25). According to these findings, the DNAm landscape may be able to distinguish between the many subtypes of IBD and the severity of the disease. Unlike genetic biomarkers, DNAm profile could cover the effects stemmed from environment and age. Additionally, disease-associated DNAms are typically universal, and these markers tend to be stable in bloodstream and tissues. Therefore, designing multiple methylation markers detection panels could potentially become an effective way to diagnose IBD in clinical practices (26).
Other epigenetic mechanisms
RNA methylation: N6-methyladenosine (m6A) refers to the methylation occurring at the sixth N of adenine base of RNA (27,28). It is assumed that SNPs located close to or inside of m6A motifs (m6A-SNPs) could be potential factors contributing to the pathology of several diseases. Several genes harboring m6A-SNPs were also reported to differentially expressed in IBD patients compared to healthy controls (i.e., UBE2L3 and SLC22A4 for CD and TCF19, C6orf47 and SNAPC4 for UC) (29).
Histone modification: histone could be modified by specific enzymes following numerous ways, including acetylation, methylation, phosphorylation, and ubiquitination. Based on the targeted amino acid (e.g., lysine, arginine, serine, threonine, tyrosine), type of histone modification and its level (number of modifying groups added) could result in a different impact (permissive/repressive) on transcriptional activity (30-32). A lot of studies have identified the correlation between differential histone acetylation pattern and the alterations of microbial composition in gut microbiota, which was documented to be related with the pathogenesis of IBD (33).
Non-coding RNAs: among various non-coding RNA types, miRNA (miR) has been studied more thoroughly due to its participation in the process of regulating the interaction of immune cells, intestinal epithelial barrier, and the homeostasis between host and intestinal microbiome. By identifying a up-regulation of miR-21 and miR-92a in UC blood, a method utilizing these two indicators was proposed, this method was able to discriminate UC from healthy subjects and irritable bowel syndrome (IBS) with an AUC of 0.979 and 0.844, respectively (34). At a specific cut-off value of 1.52 for miR-21 and 1.66 for miR-92a, the method showed a sensitivity of 87.5% and the specificity of 91.7% and 87.5% (34). Moreover, many other miRNA molecules were also reported to be correlated with the activity stage and prognosis of CD and UC such as miR-375 and miR-146 (35-37). Additionally, based on the significant correlation between disease activity of UC and miR-233 expression, an approach proposed by Schönauen et al. using such indicator in feces could well discriminate active IBD patients from subjects in remission stage with the sensitivity of 80% and the specificity of 93% (38). miR-1307-3p, miR-3615 and miR-4792 in CD4+ and CD8+ T cells were also discovered to be the potential markers to predict the prognosis of IBD (39). Besides, another interest of research has been the relationship between miRNA and the response of IBD patients to therapies. A recent study (40) reported changes of 5 candidate miRNAs (i.e., miR-126, let-7c, miR-146a, miR-146b, and miR-320a) that were associated with mucosal inflammation and clinical response to anti-TNF- agents and glucocorticoids.
Epigenetic studies in Vietnam
The advent of various cutting-edge wet-lab technologies as well as analytic algorithms enabled the research in the epigenetic field to move on faster. It is possible to discover complex mechanisms underlying human diseases, and explain the impact of many biomarkers on their pathogenesis. Subsequently, by utilizing this understanding, novel methods for treatment and diagnosis was promising to tackle health issues.
Epigenetics in Vietnam has been studied in several groups, especially groups at National Cancer Hospital. Nguyen et al. [2019] investigated EGFR methylation alteration in lung adenocarcinomas with mutations of BRCA1, MGMT, and RASSF1A (41). Ta et al. [2020] studied the pathway mechanism related to RAS/RAF mutations and epigenetic alterations in colorectal cancer (42). However, till now there is no standardized DNAm pipeline and case studies in IBD dataset. Most of the studies on IBD in Vietnam were conducted in a single center with small sample size (less than 100) and focused on clinical symptoms, endoscopic findings, and role of some non-invasive tests such as fecal calprotectin (43,44). However, the gap in the understanding of this disease from different specialties’ perspectives has been reported. One study by Dao et al. showed that the agreement between endoscopic findings and histopathology results were only 26.5% while after the revision from the expert, the confirmed diagnosis reached 49.1% (45). The lack of national data on prevalence and burden to the healthcare system as well as the lack of connection among different specialties including gastroenterology, histopathology, dietitian, surgeons or between clinical settings and translational research has been seen (45). From the overall situation, the association between DNAm and IBD thus lacks comprehensive investigation in Vietnam. In current work, we aim to propose a DNAm analysis pipeline based on deep learning with potential application to IBD and its interaction with the available pharmaceutical armamentarium to reduce the cost of treatment of IBD is of great interest in a developing country like Vietnam.
Methods
We aimed to develop a progress analysis based on the architecture of MethylNet framework (46). Our pipeline was built to get inputs from any sequencing platforms and easy to config for users.
Figure 1 illustrated a pipeline for analyzing methylation data from different data platforms such as the Methylation EPIC chip [450K or 850K from Illumina (47)] and the sequencing data (from PacBio HiFi Seq). All input from platforms is processed for further analysis steps by converting input into a beta matrix containing methylation level which is the ratio of intensities between methylated and unmethylated alleles with a range from 0 to 1 where 0 is unmethylated and 1 is fully methylated. The genomic sequence data from PacBio Seq is aligned from Fastq to Bam and methylation information is extracted. Methylation information combines CpG island site (18) in order to create a beta matrix in the annotation step in Figure 2. On the other hand, data from chip methylation is preprocessed by PymethylProcess with quality control, functional normalization, sex and SNP removal, imputation, and Feature selection. After that, it is possible to harmonize the beta matrices generated from the different sources into a single matrix to undergo further analysis.
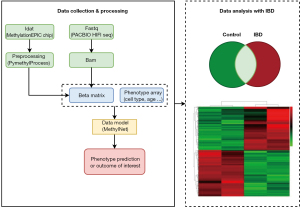
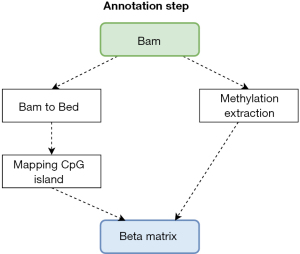
Beta matrix and phenotype, the outcome of interest, are leveraged to train a model called MethylNet (46) and discussed in the next section. MethylNet is chosen for the main tool in the pipeline to explore the beta matrix of DNAm due to several points: (I) end-to-end training method that performs predictions based on the derived features and extracts physiologically significant aspects through latent encoding; (II) the output forecasts for multiple targets regression tasks, like cell-type deconvolution and subject age forecasting. Finally, data analysis with IBD dataset includes differential expression analysis and case-control study will be driven depending on availability of the dataset to get a better understanding of the disease mechanism.
Results
There are numerous factors involved in the development and progression of IBD, including age and cell types in the immune system. Ruel et al. [2014] found that there are changes of biological networks in the demographics and phenotype of IBD in different ages (48). Another study pointed out that immunological factors and therapies in the innate immune system are important in the pathogenesis of IBD (49). Therefore, to create a control panel for IBD, we evaluated a blood DNAm dataset from Johansson public data GSE87571, which contains age and cell type information. This is one of largest public DNAm datasets which has an age range between 15 and 95 years, the number of samples is n=732 (approximately 80% for training and 20% for testing) and the chip is MethylationEPIC 450K from Illumnia (50). We used our pipeline for preprocessing data and the pretrain MethylNet model to predict the chronological age of each sample. Predicted age of this model witnessed significant correlation to ground truth (R2=0.96, P<0.001) (Figure 3A).
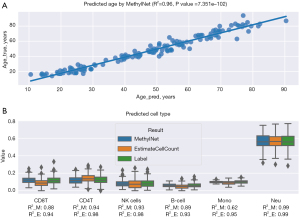
Moreover, reference-based cell type proportions were estimated by using a library of cell specific leukocyte differentially methylated regions (L-DMR). Hundred CpG features was well-established to possibly predict cell type on different methods precisely (51). In this task, we passed the data to our pipeline and used estimateCellCount2, and Methylnet as a basic library to estimate cell type data: B-cell, CD4T, CD8T, monocytes (Mono), natural killer (NK) cells, and neutrophils (Neu). This tool is an implementation of the Houseman (18) regression calibration approach algorithm to the Illumina 450K microarray for deconvoluting heterogeneous tissue sources like blood (Figure 3B). Two approaches showed a significant correlated results in distribution of cell type between the estimation and data from label with the highest R2=0.99 in Neu group for both, and the lowest R2 presented in Mono cell for pipeline with Methylnet model and B cell for estimateCellCount with R2 of 0.62 and 0.93, respectively. With these positive results, we expected this pipeline could be a foundation for the development of a bioinformatic tool able to make early diagnosis and distinguish major forms of IBD in Vietnam by using methylation data.
Discussion
IBD is defined as a chronic inflammation of digestive tract mucosa resulting in many symptoms such as diarrhea, rectal bleeding, abdominal pain, fatigue, and weight loss. Despite the fact that certain people’s risk of having IBD is undoubtedly influenced by genetic variations, it is well-known that the human genome has remained unchanged over generations, thus, the significant increase in IBD occurrences in recent decades could be considered as obvious evidence supporting a critical role of the epigenetics in IBD pathogenesis. Moreover, many of the risk factors of IBD including race or ethnicity, family history, age, cigarette smoking, and nonsteroidal anti-inflammatory medications are also major epigenetic factors (52).
Alterations of DNAm patterns have been well-documented to be associated with development and progression of various traits and disorders. The advent of PacBio sequencing technology has provided a complete solution which not only offered genome DNA sequence with highly accurate long read data, but also simultaneously brought about considerable methylation data. Therefore, our DNAm analysis pipeline was created to possibly handle types of data from this new sequencing technology. Moreover, by analysing the public blood DNAm dataset using our pipeline, we confirmed the ability of age prediction (R2=0.96) and cell type deconvolution (highest R2=0.99) which are two key factors contributing to IBD pathogenesis.
DNAm detection in IBD patients can have potential for early diagnosis and prognosis, as well as to screen for colorectal cancer. While the gold standard for diagnosing IBD is combination of clinical symptoms, endoscopic findings and histopathology features, there are still barriers in confirming diagnosis and following up patients. Colonoscopy is an invasive method, unfeasible for monitoring during treatment and requires experienced performance for good practice. The development of liquid biopsy and DNAm detection for IBD which were described in previous studies (19,23,24), therefore, can provide novel non-invasive methods for the detection of both subtypes of IBD, UC and CD. Furthermore, several studies reported promising results in using DNAm for colorectal cancer screening in IBD patients (53-55). Especially in coronavirus disease 2019 (COVID-19) pandemic, the role of non-invasive methods and point-of-care approaches are becoming more and more important.
The strategy in IBD management is to relieve symptoms by reducing the inflammation in gastrointestinal tract mucosa and help to slow the progression of the disease. Recently, a new class of drugs, called biologic agents, which are antibodies that are given to IBD patients to help their immune cells fight the inflammation; in addition to the immunomodulators which are also used to damp down the immune system’s inflammatory response (56). Several post-hoc analyses of early introduction of immunosuppressive and biologic therapy suggest the benefit for both short-term and long-term outcome for IBD patients, especially for CD patients [e.g., clinical remissions, mucosal healing, and normal C-reactive protein (CRP)] (57-61). Hence, the new detection approach of IBD using DNAm-based liquid biopsy can contribute to improve the therapeutic outcome and decrease the risk of serious adverse events. Besides, the changes of diet after diagnosis are also an important factor in managing flare-ups of both diseases (62). Taken together, the earlier the detection of IBD, the higher chance of IBD curation (63).
In summary, the application of our DNAm pipeline in IBD research is expected to improve the understanding of the impact of epigenetics in IBD and provide a potential non-invasive approach for screening and early diagnosis of IBD and furthermore, can be low-cost and effective in clinical settings. For the next step, we would like to recruit IBD Vietnamese samples to (I) validate the appropriateness and the accuracy of the deep learning models on the Vietnamese population; (II) improve the pipeline to optimize the specificity and sensitivity for the diagnosis application. The on-going GIVES-21 study recruits patients with the follow-up for 6 months to confirm diagnosis and comprehensive data (clinical symptoms, severity grades in both endoscopy and histopathology, environmental factors etc.) as well as biospecimens (biopsy tissue, blood, stool). The standardized protocol in recruitment and multi-centre design will be helpful to build up the IBD dataset in Vietnam (5).
Strengths and limitations
Strengths: By understanding the significant association and potential application of epigenetic mechanisms including DNA and RNA methylation, histone modifications, short and long non-coding RNAs in IBD research as well as clinical practice, we desired to propose a publicly free DNAm analysis pipeline compatible with all currently available data generateing platforms. Our pipeline was able to accurately perform on two datasets of age prediction and cell type deconvolution, and was promising for applications in IBD diagnosis and prognosis.
Limitations: In spite of high accuracy in public dataset, the major drawback of this study was the deficiency of methylation dataset of IBD patients for the validation of our novel model. However, our on-going project with the purpose of recruitment and building up IBD dataset to accelerate the process of developing a new diagnostic method using methylation pattern.
Conclusions
DNAm is the most studied epigenetic change and its correlation to IBD pathogenesis has been established. Our proposed DNAm pipeline was a potential foundation for the development of a screening and early diagnosis approach by using epigenetic information in Vietnam.
Acknowledgments
Funding: None.
Footnote
Provenance and Peer Review: This article was commissioned by the Guest Editors (Patrick Varga-Weisz and Raquel Franco Leal) for the series “Evidence of Epigenetics in Inflammatory Bowel Diseases” published in Digestive Medicine Research. The article has undergone external peer review.
Data Sharing Statement: Available at https://dmr.amegroups.com/article/view/10.21037/dmr-22-82/dss
Peer Review File: Available at https://dmr.amegroups.com/article/view/10.21037/dmr-22-82/prf
Conflicts of Interest: All authors have completed the ICMJE uniform disclosure form (available at https://dmr.amegroups.com/article/view/10.21037/dmr-22-82/coif). The series “Evidence of Epigenetics in Inflammatory Bowel Diseases” was commissioned by the editorial office without any funding or sponsorship. T.H.H., V.C.D., and G.M.V. are the employee of Vingroup Big Data Institute and GeneStory JSC. T.L.P. and T.K.N. are the employee of GeneStory JSC. The authors have no other conflicts of interest to declare.
Ethical Statement: The authors are accountable for all aspects of the work in ensuring that questions related to the accuracy or integrity of any part of the work are appropriately investigated and resolved.
Open Access Statement: This is an Open Access article distributed in accordance with the Creative Commons Attribution-NonCommercial-NoDerivs 4.0 International License (CC BY-NC-ND 4.0), which permits the non-commercial replication and distribution of the article with the strict proviso that no changes or edits are made and the original work is properly cited (including links to both the formal publication through the relevant DOI and the license). See: https://creativecommons.org/licenses/by-nc-nd/4.0/.
References
- Coward S, Clement FM, Williamson TS, et al. The Rising Burden of Inflammatory Bowel Disease in North America from 2015 to 2025: A Predictive Model: 1959. Am J Gastroenterol 2015;110:S829. [Crossref]
- Ng SC, Shi HY, Hamidi N, et al. Worldwide incidence and prevalence of inflammatory bowel disease in the 21st century: a systematic review of population-based studies. Lancet 2017;390:2769-78. [Crossref] [PubMed]
- Ng SC, Tang W, Ching JY, et al. Incidence and phenotype of inflammatory bowel disease based on results from the Asia-pacific Crohn's and colitis epidemiology study. Gastroenterology 2013;145:158-165.e2. [Crossref] [PubMed]
- Abraham C, Cho JH. Inflammatory bowel disease. N Engl J Med 2009;361:2066-78. [Crossref] [PubMed]
- Chinese University of Hong Kong, University of Calgary. Global IBD Visualization of Epidemiology Studies (GIVES) in the 21st Century. 2022 November; Available online: https://ClinicalTrials.gov/show/NCT04748627
- Jostins L, Ripke S, Weersma RK, et al. Host-microbe interactions have shaped the genetic architecture of inflammatory bowel disease. Nature 2012;491:119-24. [Crossref] [PubMed]
- Liu JZ, van Sommeren S, Huang H, et al. Association analyses identify 38 susceptibility loci for inflammatory bowel disease and highlight shared genetic risk across populations. Nat Genet 2015;47:979-86. [Crossref] [PubMed]
- Ramos GP, Papadakis KA. Mechanisms of Disease: Inflammatory Bowel Diseases. Mayo Clin Proc 2019;94:155-65. [Crossref] [PubMed]
- Andoh A, Imaeda H, Aomatsu T, et al. Comparison of the fecal microbiota profiles between ulcerative colitis and Crohn's disease using terminal restriction fragment length polymorphism analysis. J Gastroenterol 2011;46:479-86. [Crossref] [PubMed]
- Joossens M, Huys G, Cnockaert M, et al. Dysbiosis of the faecal microbiota in patients with Crohn's disease and their unaffected relatives. Gut 2011;60:631-7. [Crossref] [PubMed]
- Weinhold B. Epigenetics: the science of change. Environ Health Perspect 2006;114:A160-7. [Crossref] [PubMed]
- Mazzone R, Zwergel C, Artico M, et al. The emerging role of epigenetics in human autoimmune disorders. Clin Epigenetics 2019;11:34. [Crossref] [PubMed]
- Ilango S, Paital B, Jayachandran P, et al. Epigenetic alterations in cancer. Front Biosci (Landmark Ed) 2020;25:1058-109. [Crossref] [PubMed]
- Shi Y, Zhang H, Huang S, et al. Epigenetic regulation in cardiovascular disease: mechanisms and advances in clinical trials. Signal Transduct Target Ther 2022;7:200. [Crossref] [PubMed]
- Christensen BC, Houseman EA, Marsit CJ, et al. Aging and environmental exposures alter tissue-specific DNA methylation dependent upon CpG island context. PLoS Genet 2009;5:e1000602. [Crossref] [PubMed]
- Bell CG, Lowe R, Adams PD, et al. DNA methylation aging clocks: challenges and recommendations. Genome Biol 2019;20:249. [Crossref] [PubMed]
- Titus AJ, Gallimore RM, Salas LA, et al. Cell-type deconvolution from DNA methylation: a review of recent applications. Hum Mol Genet 2017;26:R216-24. [Crossref] [PubMed]
- Houseman EA, Accomando WP, Koestler DC, et al. DNA methylation arrays as surrogate measures of cell mixture distribution. BMC Bioinformatics 2012;13:86. [Crossref] [PubMed]
- Karatzas PS, Mantzaris GJ, Safioleas M, et al. DNA methylation profile of genes involved in inflammation and autoimmunity in inflammatory bowel disease. Medicine (Baltimore) 2014;93:e309. [Crossref] [PubMed]
- Häsler R, Feng Z, Bäckdahl L, et al. A functional methylome map of ulcerative colitis. Genome Res 2012;22:2130-7. [Crossref] [PubMed]
- Nimmo ER, Prendergast JG, Aldhous MC, et al. Genome-wide methylation profiling in Crohn's disease identifies altered epigenetic regulation of key host defense mechanisms including the Th17 pathway. Inflamm Bowel Dis 2012;18:889-99. [Crossref] [PubMed]
- Cooke J, Zhang H, Greger L, et al. Mucosal genome-wide methylation changes in inflammatory bowel disease. Inflamm Bowel Dis 2012;18:2128-37. [Crossref] [PubMed]
- Adams AT, Kennedy NA, Hansen R, et al. Two-stage genome-wide methylation profiling in childhood-onset Crohn's Disease implicates epigenetic alterations at the VMP1/MIR21 and HLA loci. Inflamm Bowel Dis 2014;20:1784-93. [Crossref] [PubMed]
- Ventham NT, Kennedy NA, Adams AT, et al. Integrative epigenome-wide analysis demonstrates that DNA methylation may mediate genetic risk in inflammatory bowel disease. Nat Commun 2016;7:13507. [Crossref] [PubMed]
- Howell KJ, Kraiczy J, Nayak KM, et al. DNA Methylation and Transcription Patterns in Intestinal Epithelial Cells From Pediatric Patients With Inflammatory Bowel Diseases Differentiate Disease Subtypes and Associate With Outcome. Gastroenterology 2018;154:585-98. [Crossref] [PubMed]
- Laird PW. The power and the promise of DNA methylation markers. Nat Rev Cancer 2003;3:253-66. [Crossref] [PubMed]
- Perry RP, Kelley DE. Existence of methylated messenger RNA in mouse L cells. Cell 1974;1:37-42. [Crossref]
- Desrosiers R, Friderici K, Rottman F. Identification of methylated nucleosides in messenger RNA from Novikoff hepatoma cells. Proc Natl Acad Sci U S A 1974;71:3971-5. [Crossref] [PubMed]
- Sebastian-delaCruz M, Olazagoitia-Garmendia A, Gonzalez-Moro I, et al. Implication of m6A mRNA Methylation in Susceptibility to Inflammatory Bowel Disease. Epigenomes 2020;4:16. [Crossref] [PubMed]
- Agricola E, Verdone L, Di Mauro E, et al. H4 acetylation does not replace H3 acetylation in chromatin remodelling and transcription activation of Adr1-dependent genes. Mol Microbiol 2006;62:1433-46. [Crossref] [PubMed]
- Gansen A, Tóth K, Schwarz N, et al. Opposing roles of H3- and H4-acetylation in the regulation of nucleosome structure––a FRET study. Nucleic Acids Res 2015;43:1433-43. [Crossref] [PubMed]
- Kurdistani SK, Tavazoie S, Grunstein M. Mapping global histone acetylation patterns to gene expression. Cell 2004;117:721-33. [Crossref] [PubMed]
- Lukovac S, Belzer C, Pellis L, et al. Differential modulation by Akkermansia muciniphila and Faecalibacterium prausnitzii of host peripheral lipid metabolism and histone acetylation in mouse gut organoids. mBio 2014;5:e01438-14. [Crossref] [PubMed]
- Ahmed Hassan E, El-Din Abd El-Rehim AS, Mohammed Kholef EF, et al. Potential role of plasma miR-21 and miR-92a in distinguishing between irritable bowel syndrome, ulcerative colitis, and colorectal cancer. Gastroenterol Hepatol Bed Bench 2020;13:147-54. [PubMed]
- Alam KJ, Mo JS, Han SH, et al. MicroRNA 375 regulates proliferation and migration of colon cancer cells by suppressing the CTGF-EGFR signaling pathway. Int J Cancer 2017;141:1614-29. [Crossref] [PubMed]
- Garo LP, Ajay AK, Fujiwara M, et al. MicroRNA-146a limits tumorigenic inflammation in colorectal cancer. Nat Commun 2021;12:2419. [Crossref] [PubMed]
- Wang JP, Dong LN, Wang M, et al. MiR-146a regulates the development of ulcerative colitis via mediating the TLR4/MyD88/NF-κB signaling pathway. Eur Rev Med Pharmacol Sci 2019;23:2151-7. [PubMed]
- Schönauen K, Le N, von Arnim U, et al. Circulating and Fecal microRNAs as Biomarkers for Inflammatory Bowel Diseases. Inflamm Bowel Dis 2018;24:1547-57. [Crossref] [PubMed]
- Kalla R, Adams AT, Ventham NT, et al. Whole Blood Profiling of T-cell-Derived microRNA Allows the Development of Prognostic models in Inflammatory Bowel Disease. J Crohns Colitis 2020;14:1724-33. [Crossref] [PubMed]
- Batra SK, Heier CR, Diaz-Calderon L, et al. Serum miRNAs Are Pharmacodynamic Biomarkers Associated With Therapeutic Response in Pediatric Inflammatory Bowel Disease. Inflamm Bowel Dis 2020;26:1597-606. [Crossref] [PubMed]
- Nguyen QN, Vuong LD, Truong VL, et al. Genetic and epigenetic alterations of the EGFR and mutually independent association with BRCA1, MGMT, and RASSF1A methylations in Vietnamese lung adenocarcinomas. Pathol Res Pract 2019;215:885-92. [Crossref] [PubMed]
- Ta TV, Nguyen QN, Chu HH, et al. RAS/RAF mutations and their associations with epigenetic alterations for distinct pathways in Vietnamese colorectal cancer. Pathol Res Pract 2020;216:152898. [Crossref] [PubMed]
- Nguyen GHT. Khảo sát nồng độ calprotectin trong phân ở bệnh nhân viêm loét đại trực tràng chảy máu. Hanoi: Hanoi Medical University; 2020.
- Nguyen DL, Nguyen TVH. Đặc điểm lâm sàng và cận lâm sàng của bệnh Crohn trẻ em tại Bệnh viện Nhi trung ương. Vietnam Medical Journal 2022. doi:
10.51298/vmj.v519i2.3607 .10.51298/vmj.v519i2.3607 - Dao H, Vu SV, Tran TTT, et al. Đánh giá độ phù hợp giữa kết quả mô bệnh học và đặc điểm nội soi trong chẩn đoán bệnh viêm ruột mạn tính. Vietnam Medical Journal 2021. doi:
10.51298/vmj.v499i1-2.261 .10.51298/vmj.v499i1-2.261 - Levy JJ, Titus AJ, Petersen CL, et al. MethylNet: an automated and modular deep learning approach for DNA methylation analysis. BMC Bioinformatics 2020;21:108. [Crossref] [PubMed]
- Moran S, Arribas C, Esteller M. Validation of a DNA methylation microarray for 850,000 CpG sites of the human genome enriched in enhancer sequences. Epigenomics 2016;8:389-99. [Crossref] [PubMed]
- Ruel J, Ruane D, Mehandru S, et al. IBD across the age spectrum: is it the same disease? Nat Rev Gastroenterol Hepatol 2014;11:88-98. [Crossref] [PubMed]
- Lee SH, Kwon JE, Cho ML. Immunological pathogenesis of inflammatory bowel disease. Intest Res 2018;16:26-42. [Crossref] [PubMed]
- Johansson A, Enroth S, Gyllensten U. Continuous Aging of the Human DNA Methylome Throughout the Human Lifespan. PLoS One 2013;8:e67378. [Crossref] [PubMed]
- Salas LA, Koestler DC, Butler RA, et al. An optimized library for reference-based deconvolution of whole-blood biospecimens assayed using the Illumina HumanMethylationEPIC BeadArray. Genome Biol 2018;19:64. [Crossref] [PubMed]
- Zhang YZ, Li YY. Inflammatory bowel disease: pathogenesis. World J Gastroenterol 2014;20:91-9. [Crossref] [PubMed]
- Kisiel JB, Klepp P, Allawi HT, et al. Analysis of DNA Methylation at Specific Loci in Stool Samples Detects Colorectal Cancer and High-Grade Dysplasia in Patients With Inflammatory Bowel Disease. Clin Gastroenterol Hepatol 2019;17:914-921.e5. [Crossref] [PubMed]
- Tang D, Liu J, Wang DR, et al. Diagnostic and prognostic value of the methylation status of secreted frizzled-related protein 2 in colorectal cancer. Clin Invest Med 2011;34:E88-95. [Crossref] [PubMed]
- Tham C, Chew M, Soong R, et al. Postoperative serum methylation levels of TAC1 and SEPT9 are independent predictors of recurrence and survival of patients with colorectal cancer. Cancer 2014;120:3131-41. [Crossref] [PubMed]
- Danese S, Vuitton L, Peyrin-Biroulet L. Biologic agents for IBD: practical insights. Nat Rev Gastroenterol Hepatol 2015;12:537-45. [Crossref] [PubMed]
- Colombel JF, Sandborn WJ, Rutgeerts P, et al. Adalimumab for maintenance of clinical response and remission in patients with Crohn's disease: the CHARM trial. Gastroenterology 2007;132:52-65. [Crossref] [PubMed]
- Kwak MS, Kim DH, Park SJ, et al. Efficacy of early immunomodulator therapy on the outcomes of Crohn's disease. BMC Gastroenterol 2014;14:85. [Crossref] [PubMed]
- Safroneeva E, Vavricka SR, Fournier N, et al. Impact of the early use of immunomodulators or TNF antagonists on bowel damage and surgery in Crohn's disease. Aliment Pharmacol Ther 2015;42:977-89. [Crossref] [PubMed]
- Schreiber S, Colombel JF, Bloomfield R, et al. Increased response and remission rates in short-duration Crohn's disease with subcutaneous certolizumab pegol: an analysis of PRECiSE 2 randomized maintenance trial data. Am J Gastroenterol 2010;105:1574-82. [Crossref] [PubMed]
- Schreiber S, Reinisch W, Colombel JF, et al. Subgroup analysis of the placebo-controlled CHARM trial: increased remission rates through 3 years for adalimumab-treated patients with early Crohn's disease. J Crohns Colitis 2013;7:213-21. [Crossref] [PubMed]
- Hou JK, Lee D, Lewis J. Diet and inflammatory bowel disease: review of patient-targeted recommendations. Clin Gastroenterol Hepatol 2014;12:1592-600. [Crossref] [PubMed]
- Knight-Sepulveda K, Kais S, Santaolalla R, et al. Diet and Inflammatory Bowel Disease. Gastroenterol Hepatol (N Y) 2015;11:511-20. [PubMed]
Cite this article as: Dao HV, Duong VC, Dao LV, Hoang LB, Nguyen TK, Pham TL, Vu GM, Hoang TH. A promising DNA methylation analysis pipeline for epigenetic studies and clinical implementation in inflammatory bowel disease. Dig Med Res 2023;6:23.