Radiomics analysis in gastrointestinal imaging: a narrative review
Introduction
In recent years, medical imaging field has found new strategies to overcome its limitations related to uncertainties in the response to therapy and prognostic evaluation thanks to the implementation of new quantitative tools able to achieve tissue characterization with a more precise and deeper approach than just visual assessment.
The advent of genomic in the early 1990s established that diseases have specific genetic fingerprints; therefore, medical research is striving to deepen the knowledge of human diseases on a genomic level, aiming at providing tailored therapeutic strategies targeting the specific genetic makeup of a pathology (1,2).
The “omics” revolution has also involved the field of medical imaging, through radiomics (3,4). Such technology aims at extracting quantitative features from medical images to improve disease characterization. Therefore, radiomics is a new emerging imaging field consisting of an automated high-yield extraction of huge amount of quantitative features, with the capability to obtain a non-invasive analysis of tumoral heterogeneity in order to extract quantitative imaging biomarkers that correlate with clinical outcome (5-7), with the ultimate aim of exploiting personalized medicine (2,8-11).
Radiomics steps includes the identification of a vast amount of quantitative features within digital images, the storage of these data in federated databases (consisting of a system in which several independent databases function as a single entity), and the subsequent data mining for knowledge extraction and application (1) (Figure 1). Many quantitative features have been already extracted using high-throughput computing from medical images such as computed tomography (CT), magnetic resonance (MR), positron emission tomography (PET), and ultrasound (US) (12-14).
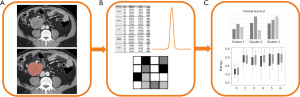
Computed tomographic texture analysis has emerged as one of the most important biomarkers able to assess tumor heterogeneity. This heterogeneity, which is characterized both at the genetic level, in cell density, angiogenesis, and necrosis, appears to be related to prognosis and treatment. Texture analysis is a part of radiomics, which involves the extraction, analysis, and inter-prediction of quantitative features from medical images (15,16).
The aim of this review article is to present the main radiomics application in gastrointestinal imaging and the potential role, limitations, and future prospective of these new biomarkers in oncologic and non-oncologic patient management. We present this article in accordance with the Narrative Review reporting checklist (available at https://dmr.amegroups.com/article/view/10.21037/dmr-22-52/rc).
Methods
Search strategy
PubMed databases were queried for primary articles and reviews in English from January 2013 to July 2022 using the search terms “radiomic”, “radiomics”, “liver”, “small bowel”, “colon”, “GI tract”, and “gastrointestinal imaging” (Table 1). Articles were included based on authors’ discretion.
Table 1
Items | Specification |
---|---|
Date of search | 20 July, 2022 |
Databases and other sources searched | PubMed |
Search terms used | Radiomic, radiomics, liver, small bowel, colon, GI tract, and gastrointestinal imaging |
Timeframe | January 2013–July 2022 |
Inclusion and exclusion criteria | Inclusion: reviews, primary studies, randomized trials, full text available, in English |
Exclusion: non-English, case report | |
Selection process | A Del Gaudio conducted the selection; D De Santis verified sources |
Any additional considerations, if applicable | References of selected articles were reviewed and those deemed relevant from title review, were included if full text was available and in English |
GI, gastrointestinal.
Colorectal cancer
Colorectal cancer is the second cause of cancer-related mortality worldwide (17), and more than 70% of patients diagnosed with locally advanced rectal cancer (LARC) (18). Preoperative chemoradiotherapy (CRT) prior to total mesorectal excision (TME) has been a standard treatment strategy. Neoadjuvant treatment reduces the risk of local recurrence and downstages the tumor. Wait-and-see strategy is gradually gaining consensus as a viable therapeutic option due to the fact that approximately 30% of subjects reach pathological complete response (pCR) (19). Texture analysis starts with cancer segmentation (Figure 2), has been applied to CT and MRI aiming at predicting long-term survival in patients with LARC; texture analysis is also able to discriminate between different cancer stages and to predict response to CRT (20). Caruso and colleagues investigated Haralick texture feature during pre-treatment MRI examination in patients affected by rectal cancer and 5 over 192 computed Haralick texture showed significant difference between non responder (NR) and complete responder (CR) (21). The same group of investigators also developed an automatic segmentation algorithm to extract radiomic features from tumor volume based on a convolutional neural network architecture (22,23). Giannini et al. evaluated texture features extracted from pre-therapy PET and MRI datasets and tested their capability to predict response to CRT/RT, aiming at personalizing patient treatment (e.g., to avoid neoadjuvant therapy toxicity in patients predicted as non-responders). A multiparametric analysis was performed and a logistic regression model formed by six second-order texture features (five from PET and one from T2-weighted MRI sequences) yielded the highest predictive performance in differentiating between responders and non-responders [area under the curve (AUC) =0.86; sensitivity =86%, and specificity =83%] (24).
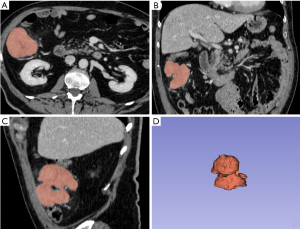
Despite a constant of reduction of local recurrence rate of rectal cancer has been significantly reduced to 5–10%, at the time of diagnosis approximately 20% to 30% of rectal cancer patients have metastatic disease; furthermore most rectal cancer deaths are related to metastatization. Liu et al. investigated the value of radiomics based on MRI to predict preoperative synchronous distant metastasis in patients with rectal cancer (25). One hundred and seventy-seven patients were enrolled (123 patients in the training cohort and 54 in the validation cohort) and a total of 385 radiomics features were extracted from pre-treatment MRI. The combined model (radiomic and clinical) outperformed the pure clinical model (AUC: 0.827; sensitivity: 72.2%, specificity: 94.4%, and accuracy: 87.0%) showing potential to be used as a noninvasive biomarker for the identification of patients at high risk of synchronous distant metastases. Similarly, Nie et al. supported the improved predictive value of multiparametric MRI features for pathologic response after preoperative CRT in patients with LARC. Forty-eight patients with non-metastatic MRI-staged T3–T4 rectal cancer underwent preoperative chemoradiation and TME surgery; volume-averaged and voxelized analysis were used to evaluate specific relationship between pre-CRT MRI data and pathologic response; by combining all categories of image information together, the final AUC improved to 0.89 (26).
Rao et al. compared texture analysis to RECIST criteria in the assessment of response to chemotherapy in a cohort of 21 patients pre- and post-chemotherapy, reporting that morphology changes in metastases used in RECIST were outperformed by quantitative changes in entropy and uniformity in discriminating between good and poor responders (27).
Pancreatic cancer
Pancreas is an organ affected by different form of neoplasms, with a large variety in terms of onset, symptoms, malignancy, response to therapy, and overall survival (OS). Even though pancreatic neoplasm are easy to detect on conventional CT and MR imaging, the assessment through these modalities of their malignancy and the consequent prediction of response to therapy is not straightforward (28,29). For such reason, several imaging biomarker are under investigation, such as diffusion-weighted imaging, perfusion CT (28,30-32), and radiomics (33,34). In this contest, radiomics showed interesting results. Some studies assessed the role of radiomics in intraductal papillary mucinous neoplasms (IPMNs) for the recognition of their malignant potential. In fact, it has been estimated that up to 82% of patients underwent surgery for over-staged benign lesions, due to the limitation of visual assessment in the risk stratification (35).
An initial approach at the problem has been proposed by Hanania and colleagues (33), who retrospectively assessed 53 CT scans of patients with IPMNs. The authors identified 14 second order texture analysis imaging biomarkers that outperformed Fukuoka criteria (AUC: 0.82, sensitivity: 85%, and specificity: 68%) in the prediction of histopathological grade. A based-model approach was also tested in other two studies (35,36); in fact, as Permuth et al. showed, a combined-model among texture parameters derived from pre-operative CT scans associated with matched plasma-based miRNA genomic classifier (MGC) predicts IPMN aggressiveness with higher accuracy than standard “worrisome” radiologic features, with an AUC of 0.92 and higher sensitivity, specificity, positive predictive value, and negative predictive value than other models alone. Similar results, confirmed in a larger patient cohort, were obtained by Chakraborty and colleagues (35). Thus, these proof-of-concept studies suggest that a non-invasive radiogenomic approach may be more accurate in the prediction of IPMNs pathology than simple radiologic and or histologic features alone. Despite fewer studies are focused on other pancreatic cystic or solid neoplasms, their results are quite promising. As for the radiomics-based model proposed by Wei et al. (37) in the preoperative computer-aided differentiation of serous cystic pancreatic neoplasms from different cystic pancreatic neoplasms, in which authors investigated 260 patients and the diagnostic model performed precisely and robustly, showing AUC of 0.837, sensitivity of 0.667, and specificity of 0.818.
A different approach has been proposed for pancreatic adenocarcinoma, since the challenge is more focused on the prediction of response to treatment and pre-operative assessment rather than detection and characterization. Cozzi and co-authors (34) proposed a radiomic-signature for the prediction of survival and local control after stereotactic body radiation therapy in pancreatic adenocarcinoma. Thanks to their retrospective radiomic analysis (performed with second and higher orders) based on clinical target volume unenhanced CT scans, they analyzed one-hundred patients randomly divided in training and validation cohorts. Results showed a significant clinical-radiomic signature associated with OS in both training and validation groups. Significant results were also observed for disease local control. Both these parameters allowed the distinction between low-risk and high-risk patients through radiomics signature. The task of preoperative assessment of pancreatic ductal adenocarcinoma has been investigated by Rigiroli et al., who assessed the role of radiomic features in the evaluation of superior mesenteric artery (SMA) involvement. They constructed a model with five radiomic features (maximum hugging angle, maximum diameter, logarithm robust mean absolute deviation, minimum distance, and square gray level co-occurrence matrix correlation) and demonstrated its higher diagnostic yield {AUC: 0.71 [95% confidence interval (CI): 0.62, 0.79]} compared with visual radiologist analysis [AUC: 0.54 (95% CI: 0.50, 0.59); P<0.001] (29).
Radiomics, moreover, may help in differentiating mass-forming pancreatitis and autoimmune pancreatitis from pancreatic cancer, as shown by several studies (38-40). Ren et al. in their retrospective study of 109 patients demonstrated the role of texture analysis on unenhanced CT in differentiating massive pancreatitis from pancreatic ductal adenocarcinoma. Using random forest (RF) method, the four most predictive parameters (GreyLevelNonuniformity_angle90_offset1, Vox-elValueSum, HaraVariance, and ClusterProminence_All-Direction_offset1_SD) have been implemented in a diagnostic predictive model reaching high diagnostic yield (AUC: 0.84, sensitivity: 82.6%, specificity: 80.8%, and accuracy: 82.1%) (38). There results were in accordance with Park et al., (39) who performed a retrospective study on 182 patients and demonstrated radiomics features are a valid support in distinguishing pancreatitis from pancreatic ductal adenocarcinoma with an accuracy of 95.2%.
As shown by several studies, radiomics and texture analysis may also play a role in pancreatic neuroendocrine tumors (pNET) diagnosis and grading, as well as in the differential diagnosis with neuroendocrine carcinoma (NEC) (41-43). pNET and NEC are indeed two pathologically different diseases requiring specific therapy. Azoulay et al. combined morphological features and texture analysis, demonstrating that NECs tend to be larger, less dense, and more heterogenous than NETs, due to the presence of hemorrhage (43).
Due to the paucity of similar investigations, larger multicentric studies are mandatory to confirm and strengthen these results; with the hope to combine a cancer radiomic-signature in a multi-modality score, useful for the optimal management of complex patients with heterogeneous cancers.
Gastro-esophageal cancer
Gastric cancer is the third most common cause of cancer death worldwide (17). Surgical resection is the main therapeutic strategy; however, adjuvant treatments need to be considered due to the high rate of recurrence (44,45). Despite chemotherapy and initial high response rates, many patients have still very poor prognosis (45,46); therefore, a more accurate patient stratification able to better predict outcomes and response to therapy is highly advisable, and radiomics has good potential to serve this cause.
In a recent study Jiang et al. evaluated whether radiomic feature-based fluorine 18 (18F) fluorodeoxyglucose (FDG) PET imaging signatures allows prediction of disease-free survival (DFS) and OS in a total of 214 patients, analyzing 80 radiomic features for each patient (47). The DFS and OS obtained with the radiomic model were significantly higher (4.169 and 3.954, respectively; all P<0.001), concluding that the radiomic signature may predict patients who could benefit from specific chemotherapy.
Recently, Klaassen and colleagues investigated the prediction of response to chemotherapy of liver metastases in patients affected by esophagogastric cancer through a radiomic RF model which extracted 370 radiomics features per lesion from the pre-treatment CT examination (9,48). The RF-model AUC was 0.79 for partial responders and 0.87 for CRs, showing the potential value of this innovative approach in patients affected by esophagogastric cancer.
In another study, Li et al. investigated the clinical prognostic value of radiomics signature in 181 patients who had undergone complete resection of gastric cancer (49). The radiomics algorithm incorporating radiomic signature and clinic-pathological risk factors (T stage, N stage, and differentiation) outperformed clinical nomogram and radiomic signature alone providing higher predictive accuracy and showing potential for stratifying gastric cancer patients into high-risk and low-risk groups, facilitating as well personalized treatment of gastric cancer.
Lastly, radiomics may found application to differentiate responder from non-responder. In a study conducted on 49 patients with esophageal cancer, Hou and colleagues found that histogram kurtosis, histogram skewness, GLSZM long-zone emphasis, and 2 Gabor transformed parameters MSA-54 and MSE-54, (AUCs from 0.686 to 0.727), differentiated non-responders from responders by means of an artificial neural network-derived prediction model (50). Improved patient stratification is still a key task for patients with gastro-esophageal cancer and radiomics is showing promising application in this setting (51).
Gastrointestinal stromal tumor (GIST)
GISTs arise from Cajal interstitial cells and represent the most common mesenchymal tumors in the gastrointestinal tract (52,53), mostly found in the stomach (50–60%), small intestines (20–30%), colon and rectum (10%), and esophagus (5%). GISTs are clinically heterogeneous, and preoperatively evaluating the biological behavior is crucial for surgical decision-making (54,55). The evaluation of the pre-operative risks is obtained by a comprehensive evaluation of symptoms, tumor burden and CT.
Machine learning can integrate clinical factors, radiomics feature, and CT images in order to comprehensively assess and successfully support the preoperative clinical management. Chen et al. (56) reported a radiomics-based prediction nomogram for patients with GISTs investigating a total of 222 patients (130 patients in the primary cohort and 92 patients in the external validation cohort). A radiomics model was generated and resulted to have an AUC of 0.867 in the primary cohort and 0.847 in the external cohort, and in the entire cohort the AUC was 0.858. This radiomics monogram was able to predict the malignancy of GISTs, proving to be a reliable tool for the preoperative clinical decision-making process.
By conducting a retrospective study on 333 patients, Wang and colleagues aimed at building radiomics predictive models based on contrast-enhanced CT to preoperatively predict mitotic count and malignant potential of GISTs, obtaining an AUC of 0.882 in the training set and an AUC of 0.920 in the validation set, showing good predictive performance and great potential for tailored clinical decision-making process (57).
Additionally, Ning et al. introduced a structure that integrates radiomics and deep learning algorithms to classify CT images of GISTs according to their malignancy (58).
Oncologic liver diseases
Primary liver cancers, such as hepatocellular carcinoma (HCC) and intrahepatic cholangiocarcinoma (ICC), are among the leading causes of cancer-related mortality worldwide with 841,080 new cases of liver cancers in 2018 (17). The development of radiomics and machine learning has potential to identify non-invasive imaging biomarkers.
Several studies analyzed images aiming at extracting predictive or prognostic quantitative information in HCC. Kiryu et al. evaluated the role of HCC heterogeneity on patient prognosis by examining the unenhanced CT scans in 122 patients undergoing hepatectomy (59). The investigators identified several texture features and reported their impact on 5-year progression-free survival and OS. A small proportion of these features were found to be associated with survival, independently from other clinic-pathologic variables; these results highlighted the potential objective prognostic ability of these quantitative imaging features.
Recently, a radiomics signature derived from texture analysis was proposed to be a surrogate for microvascular invasion (MVI) in HCC, prediction of patient survival, and early recurrence in 215 patients (60). Twenty-one radiomics features were chosen from 300 starting features and the derived radiomics signature resulted to be a significant predictor for early recurrence in HCC, outperforming clinical variables alone for preoperative estimation of early recurrence. Similarly, in another study, Hu et al. aimed at developing an ultrasound-based radiomics score for preoperative prediction of MVI in 482 patients affected by HCC, who underwent contrast-enhanced ultrasound (61). Alpha-fetoprotein, radiomics score, and tumor size have been demonstrated independent predictors of MVI in multivariate analysis; in addition, the radiomics nomogram (based on these 3 factors) outperformed the clinical nomogram in the detection of MVI (AUC: 0.731 vs. 0.634; P=0.015), and in the decision curve analysis.
Radiomics analysis was also used to differentiate pathologic grade. Another research group analyzed 46 patients who had undergone contrast-enhanced MRI prior to tumor resection. Low-grade HCCs had higher mean intensity and decreased gray-level run-length non-uniformity in four directions. These results indicate that internal heterogeneity could represent an imaging feature related to high-grade HCC, predicting its clinical prognosis (62). In addition, another study group explored the CT-based radiomics data related to crucial genomic information, such as doxorubicin-resistant genes (TP53, TOP2A, CTNNB1, CDKN2A, and AKT1), and identified radiomics signatures that effectively differentiate these chemo-resistant genes (63).
ICC is the second most common primary liver tumor after HCC (64). Pre-operative ICC staging is routinely achieved by contrast-enhanced CT. However, many patients deemed resectable with CT are eventually found to be unresectable during surgery, a condition known as futile resection. In this regard, Chu et al. (65) constructed a combined logistic model incorporating radiomics signature and clinical risk factors for preoperative prediction of futile resection in ICC, which outperformed the clinical model in the prediction of futile resection (AUC of 0.804 vs. 0.590). Ji et al. validated a radiomics nomogram related to lymph node status and CA 19.9 in the prediction of nodal metastases in patients with ICC. This nomogram provided good diagnostic performances, especially in the subgroup of patient with CT-reported negative lymph nodes (66).
Surgery is the treatment of choice for most patients with resectable ICC, however, the postoperative prognosis is poor due to the high incidence of recurrence and metastasis (67). MVI is regarded as an effective predictor of recurrence and poor OS in patients undergoing surgical resection of ICC (68). Predicting MVI, through preoperative imaging (CT and MRI), is essential to help physicians in choosing the optimal treatment for the patient. In this regard, Zhou et al. developed a radiomics signature based on dynamic contrast-enhanced magnetic resonance imaging for preoperative prediction of MVI in patients with massive ICC. One hundred and twenty-six patients with surgically resected massive ICC (34 MVI-positive and 92 MVI-negative) were retrospectively enrolled for the study and assigned to training and validation cohorts with a 7:3 ratio. The radiomic signature, composed of seven wavelet features, obtained optimal prediction performance in both training and validation cohorts (AUCs =0.873 and 0.850, respectively), proving to be a reliable imaging biomarker for the prediction of MVI of ICC (69).
Another therapeutic strategy for ICC is trans-arterial radioembolization; however, the efficacy of this therapy depends on the architecture of the tumor (70). Mosconi et al. (71) applied texture analysis to pre-operative CT, demonstrating how ICCs most likely to be effectively treated by trans-arterial radioembolization show higher iodine uptake in the arterial phase and more homogeneous distribution of texture parameters (lower kurtosis, GLCM contrast, GLCM dissimilarity; higher GLCM homogeneity and GLCM correlation).
Radiomics applied both to CT and MRI proved also to be effective in differentiating HCC from ICC and combined HCC-ICC (72). In particular, the best prediction accuracy was obtained by histogram parameters applied to ADC maps in association with LI-RADS (73). These results might have significant clinical implications, since the liver resection varies between combined HCC-ICC and HCC (74); however, large prospective multicentric trial is still required to further validate these findings.
Non-oncologic liver diseases
The potential benefit of radiomics in the evaluation of non-oncologic liver diseases is under active investigation. Liver biopsy remains the reference standard technique in the diagnosis and staging of a wide range of liver diseases; however, different imaging modalities are being increasingly used in order to offer a valid non-invasive alternative diagnostic option to the patient. Nonetheless, all these techniques traditionally rely on subjective visual assessment; therefore, just like biopsy, they are hampered by interobserver variability. In this scenario, radiomics holds promise to guarantee an objective assessment of various liver diseases, ultimately guiding the clinical management with a patient-tailored approach.
Liver fibrosis is the main determinant of morbidity and mortality in patients with chronic liver disease (75). A timely diagnosis and an accurate staging are the fundamental preconditions for the therapeutical decision-making, in order to prevent the progression to end-stage disease (76). Nowadays, imaging and laboratory tests are often preferred to biopsy in the diagnosis and follow-up of liver fibrosis, owing to their non-invasiveness and the possibility of being easily repeated during the course of the disease.
Morphologic and parenchymal changes related to fibrosis, including surface nodularity, fibrotic septa and regenerative nodules, can be detected with conventional liver MRI; however, its accuracy and reproducibility in multiclass staging of liver fibrosis remains controversial (77,78). In a recent publication, Park et al. (79) retrospectively investigated the diagnostic yield of a model based on radiomics features derived from gadoxetic acid-enhanced hepatobiliary phase MRI datasets in differentiating between F0–F2 liver fibrosis and advanced fibrosis (F3–F4) or cirrhosis (F4), using liver biopsy as reference standard. After being validated in a development cohort, the model has been tested to calculate the radiomics fibrosis index in a population of 107 patients with pathologically proven liver fibrosis, showing high accuracy for liver fibrosis staging, outperforming both conventional MRI and serum markers, such as the aspartate transaminase-to-platelet ratio index and the fibrosis-4 index. Notably, the authors demonstrated an excellent inter-reader agreement for the radiomics fibrosis index, suggesting its promising role as non-invasive biomarker for chronic liver disease evaluation.
In recent years, encouraging results have also been obtained by applying radiomics to other imaging modalities for the study of liver fibrosis. Naganawa et al. (80) developed a model to predict the presence of nonalcoholic steatohepatitis (NASH) by using parameters extracted from texture analysis of unenhanced CT images. Authors reported that the model is able to effectively predict the presence of NASH in patients without suspicion of liver fibrosis, namely patients with normal levels of serum hyaluronic acid, with an accuracy of 92% in the validation dataset. On the other hand, however, texture analysis showed only mediocre results in predicting NASH in patients with liver fibrosis.
Two-dimensional shear wave elastography (2D-SWE) is an emerging US technique that allows the real-time measurements of liver stiffness and has a growing use in the assessment of liver fibrosis. However, several previously published studies exhibited broad variability of cut-off values for staging liver fibrosis (81,82). In a recent prospective multicenter study (83), Wang et al. evaluated the performance of a newly developed deep learning radiomics model of 2D-SWE for the staging of liver fibrosis in a cohort of patients with chronic hepatitis B, compared to liver stiffness measurement and serum biomarkers. Radiomics demonstrated results comparable to those of liver biopsy, and performed significantly better than other techniques, with an area under the ROC curve of 0.98 for advanced fibrosis and of 0.97 for cirrhosis. Moreover, radiomics analysis showed no substantial variation in its performance, provided that a sufficient number of 2D-SWE images were obtained during the training of the deep learning method.
In addition to the evaluation of liver fibrosis, radiomics analysis can be applied in patient with chronic liver disease to detect clinically significant portal hypertension, which is associated with severe complications such as portal systemic encephalopathy and bleeding esophageal varices. This approach is particularly enticing when invasive methods such as hepatic venous pressure gradient measurement, are not feasible.
Inflammatory bowel disease
Crohn’s disease is the most representative among inflammatory bowel diseases and is routinely assessed with MRI enterography, that significantly correlates with endoscopic and histologic findings (84). In fact, this imaging technique effectively evaluates mural bowel involvement (including mural thickness, contrast enhancement, and bowel morphologic irregularities) and assesses disease activity and potential complications, such as entero-enteric fistula, bowel obstruction, and transmural bowel fibrosis. As aforementioned, texture analysis has the intrinsic potential to quantify image heterogeneity of a tissue not assessable by human eye (85). Few authors started to apply this approach to inflammatory bowel disease, aiming at assessing ultrastructural tissue changes appreciable in active disease or in treatment response. Makanyanga and colleagues (86) retrospectively examined sixteen patients that underwent MRI enterography with diagnosis of Crohn’s disease before ileal resection. Small region of interests were placed on T2-weighted images and compared with the same region observed in the gross specimen and assessed with acute inflammatory scores (AIS) by the pathologist. In addition, MRI activity (including mural thickness, T2 signal, T1 enhancement) was scored with Crohn disease activity score (CDAS) and then correlated with other regions of interest (ROIs). Results showed significant correlation of skewness with AIS, while entropy and kurtosis showed significant correlation with CDAS. Moreover, entropy and mean showed significant association with T2 signal, as well as MPP correlated with mural thickness. Despite the small sample size, obtained data are encouraging and a larger patient cohort might be advisable to confirm the results. Perhaps, the most likely role of texture analysis in Crohn’s disease could be an objective non-invasive imaging biomarker of treatment response: for instance, measurable changes in skewness could act as a biomarker for reduced histological inflammation. Another interesting study proposed by Bhatnagar et al. (87) investigated the possible correlation, in patients affected by Crohn’s disease, between texture analysis and histological markers of hypoxia and angiogenesis. Seven patients who had undergone preoperative MRI enterography, were retrospectively analyzed with texture analysis placing transmural ROIs on post-contrast T1 weighted images in the ileal segments planned to be surgically removed. Histological reports of the resected bowel segments also analyzed microvessel density (MVD), staining for markers of hypoxia (HIF-1α), and angiogenesis (VEGF), essential for the estimation of disease activity. These parameters were subsequently compared with texture analysis and results showed a significant opposite correlation of mean, standard deviation and mean of positive pixels in specimen expressing VEGF. Additionally, a significant correlation was reported between skewness and MVD. These results sound promising for the possible future idea to apply texture parameters in the assessment of disease activity and, in case of medical therapy, to use them for response to therapy assessment. This possibility might avoid invasive procedures and enable the possibility to study the entire bowel in a single MRI examination. This notwithstanding, larger multi-centric studies are mandatory to obtain more results on larger patient cohorts and to explore whether texture analysis might also predict the response to treatment before the beginning of the therapy also in inflammatory bowel diseases.
Limitations
Although research in radiomics has gained momentum in recent years, some limitation should be addressed. The major limitation is the reproducibility of the multitude of imaging-derived signatures offered by radiomics, which is critical for its dissemination in clinical practice (88). The lack of reproducibility derives from differences in fixed steps involved in radiomics, such as image acquisition, segmentation, feature extraction, and selection; such differences hamper the reproducibility of results not only in multicenter studies but also in single-center investigations (89). Several phantom studies have shown how changing acquisition and reconstruction settings, such as the reconstruction algorithm, reconstruction kernel, and tube current, can reduce the percentage of reproducible radiomic features up to 43% (89). In particular, section thickness is the parameter having the deepest impact on radiomics features reproducibility. On the other hand, the radiomic features most affected by CT reconstruction settings are texture and intensity categories (including mean attenuation), while radiomic features related to i shape (such as maximum volume and axial diameter) are unaffected. Therefore, a thorough selection of radiomic features, with or without empirical correction factors, should be used to overcome these limitations (90).
Finally, to increase the reproducibility of radiomics features and make them applicable to radiology practice, it is necessary to improve the level of evidence of radiomics studies by increasing the sample size, including both positive and negative results, and ensuring the presence of external validation. In addition, is essential to involve properly trained staff members in radiomics studies and the use of open-access feature extraction software is advisable (88).
Conclusions
Radiomics presents itself as a quantitative tool potentially playing a central role in medicine, due to the growing need for a personalized patient treatment. Its integration with clinical data, pathological results, and molecular data may ultimately allow for timely and accurate diagnosis, optimal treatment strategy, and early prediction of outcomes. Several applications are currently under investigation in the field of oncological and inflammatory gastrointestinal imaging, with promising results; nevertheless, efforts should be made to strengthen its applicability by implementing strategies to improve its reproducibility.
Acknowledgments
Funding: None.
Footnote
Provenance and Peer Review: This article was commissioned by the Guest Editor (Paolo Mercantini) for the series “Multidisciplinary Treatment in Gastrointestinal Cancer” published in Digestive Medicine Research. The article has undergone external peer review.
Reporting Checklist: The authors have completed the Narrative Review reporting checklist. Available at https://dmr.amegroups.com/article/view/10.21037/dmr-22-52/rc
Conflicts of Interest: All authors have completed the ICMJE uniform disclosure form (available at https://dmr.amegroups.com/article/view/10.21037/dmr-22-52/coif). The series “Multidisciplinary Treatment in Gastrointestinal Cancer” was commissioned by the editorial office without any funding or sponsorship. The authors have no other conflicts of interest to declare.
Ethical Statement: The authors are accountable for all aspects of the work in ensuring that questions related to the accuracy or integrity of any part of the work are appropriately investigated and resolved.
Open Access Statement: This is an Open Access article distributed in accordance with the Creative Commons Attribution-NonCommercial-NoDerivs 4.0 International License (CC BY-NC-ND 4.0), which permits the non-commercial replication and distribution of the article with the strict proviso that no changes or edits are made and the original work is properly cited (including links to both the formal publication through the relevant DOI and the license). See: https://creativecommons.org/licenses/by-nc-nd/4.0/.
References
- Pinker K, Shitano F, Sala E, et al. Background, current role, and potential applications of radiogenomics. J Magn Reson Imaging 2018;47:604-20. [Crossref] [PubMed]
- Aerts HJ. The Potential of Radiomic-Based Phenotyping in Precision Medicine: A Review. JAMA Oncol 2016;2:1636-42. [Crossref] [PubMed]
- Epstein RJ, Lin FP. Cancer and the omics revolution. Aust Fam Physician 2017;46:189-93. [PubMed]
- Harries L, Goljanek-Whysall K. The biology of ageing and the omics revolution. Biogerontology 2018;19:435-6. [Crossref] [PubMed]
- Avanzo M, Stancanello J, El Naqa I. Beyond imaging: The promise of radiomics. Phys Med 2017;38:122-39. [Crossref] [PubMed]
- Rizzo S, Botta F, Raimondi S, et al. Radiomics: the facts and the challenges of image analysis. Eur Radiol Exp 2018;2:36. [Crossref] [PubMed]
- Caruso D, Polici M, Zerunian M, et al. Radiomics in Oncology, Part 2: Thoracic, Genito-Urinary, Breast, Neurological, Hematologic and Musculoskeletal Applications. Cancers (Basel) 2021;13:2681. [Crossref] [PubMed]
- Acharya UR, Hagiwara Y, Sudarshan VK, et al. Towards precision medicine: from quantitative imaging to radiomics. J Zhejiang Univ Sci B 2018;19:6-24. [Crossref] [PubMed]
- Klaassen R, Larue RTHM, Mearadji B, et al. Feasibility of CT radiomics to predict treatment response of individual liver metastases in esophagogastric cancer patients. PLoS One 2018;13:e0207362. [Crossref] [PubMed]
- Caruso D, Polici M, Rinzivillo M, et al. CT-based radiomics for prediction of therapeutic response to Everolimus in metastatic neuroendocrine tumors. Radiol Med 2022;127:691-701. [Crossref] [PubMed]
- Thattaamuriyil Padmakumari L, Guido G, Caruso D, et al. The Role of Chest CT Radiomics in Diagnosis of Lung Cancer or Tuberculosis: A Pilot Study. Diagnostics (Basel) 2022;12:739. [Crossref] [PubMed]
- Lambin P, Leijenaar RTH, Deist TM, et al. Radiomics: the bridge between medical imaging and personalized medicine. Nat Rev Clin Oncol 2017;14:749-62. [Crossref] [PubMed]
- De Cecco CN, Ciolina M, Caruso D, et al. Performance of diffusion-weighted imaging, perfusion imaging, and texture analysis in predicting tumoral response to neoadjuvant chemoradiotherapy in rectal cancer patients studied with 3T MR: initial experience. Abdom Radiol (NY) 2016;41:1728-35. [Crossref] [PubMed]
- Gillies RJ, Kinahan PE, Hricak H. Radiomics: Images Are More than Pictures, They Are Data. Radiology 2016;278:563-77. [Crossref] [PubMed]
- Lubner MG, Smith AD, Sandrasegaran K, et al. CT Texture Analysis: Definitions, Applications, Biologic Correlates, and Challenges. Radiographics 2017;37:1483-503. [Crossref] [PubMed]
- Caruso D, Polici M, Zerunian M, et al. Radiomics in Oncology, Part 1: Technical Principles and Gastrointestinal Application in CT and MRI. Cancers (Basel) 2021;13:2522. [Crossref] [PubMed]
- Bray F, Ferlay J, Soerjomataram I, et al. Global cancer statistics 2018: GLOBOCAN estimates of incidence and mortality worldwide for 36 cancers in 185 countries. CA Cancer J Clin 2018;68:394-424. [Crossref] [PubMed]
- Liu Z, Zhang XY, Shi YJ, et al. Radiomics Analysis for Evaluation of Pathological Complete Response to Neoadjuvant Chemoradiotherapy in Locally Advanced Rectal Cancer. Clin Cancer Res 2017;23:7253-62. [Crossref] [PubMed]
- Maas M, Nelemans PJ, Valentini V, et al. Long-term outcome in patients with a pathological complete response after chemoradiation for rectal cancer: a pooled analysis of individual patient data. Lancet Oncol 2010;11:835-44. [Crossref] [PubMed]
- Coppola F, Giannini V, Gabelloni M, et al. Radiomics and Magnetic Resonance Imaging of Rectal Cancer: From Engineering to Clinical Practice. Diagnostics (Basel) 2021;11:756. [Crossref] [PubMed]
- Caruso D, Zerunian M, Ciolina M, et al. Haralick's texture features for the prediction of response to therapy in colorectal cancer: a preliminary study. Radiol Med 2018;123:161-7. [Crossref] [PubMed]
- Soomro MH, Coppotelli M, Conforto S, et al. Automated Segmentation of Colorectal Tumor in 3D MRI Using 3D Multiscale Densely Connected Convolutional Neural Network. J Healthc Eng 2019;2019:1075434. [Crossref] [PubMed]
- Caruso D, Polici M, Zerunian M, et al. Radiomic Cancer Hallmarks to Identify High-Risk Patients in Non-Metastatic Colon Cancer. Cancers (Basel) 2022;14:3438. [Crossref] [PubMed]
- Giannini V, Mazzetti S, Bertotto I, et al. Predicting locally advanced rectal cancer response to neoadjuvant therapy with 18F-FDG PET and MRI radiomics features. Eur J Nucl Med Mol Imaging 2019;46:878-88. [Crossref] [PubMed]
- Liu H, Zhang C, Wang L, et al. MRI radiomics analysis for predicting preoperative synchronous distant metastasis in patients with rectal cancer. Eur Radiol 2019;29:4418-26. [Crossref] [PubMed]
- Nie K, Shi L, Chen Q, et al. Rectal Cancer: Assessment of Neoadjuvant Chemoradiation Outcome based on Radiomics of Multiparametric MRI. Clin Cancer Res 2016;22:5256-64. [Crossref] [PubMed]
- Rao SX, Lambregts DM, Schnerr RS, et al. CT texture analysis in colorectal liver metastases: A better way than size and volume measurements to assess response to chemotherapy? United European Gastroenterol J 2016;4:257-63. [Crossref] [PubMed]
- Laghi A, Zerunian M, Caruso D. How new technologies could impact on radiology diagnosis and assessment of pancreatic lesions: Future perspectives. Endosc Ultrasound 2018;7:310-3. [Crossref] [PubMed]
- Rigiroli F, Hoye J, Lerebours R, et al. CT Radiomic Features of Superior Mesenteric Artery Involvement in Pancreatic Ductal Adenocarcinoma: A Pilot Study. Radiology 2021;301:610-22. [Crossref] [PubMed]
- Yadav AK, Sharma R, Kandasamy D, et al. Perfusion CT - Can it resolve the pancreatic carcinoma versus mass forming chronic pancreatitis conundrum? Pancreatology 2016;16:979-87. [Crossref] [PubMed]
- Park MS, Klotz E, Kim MJ, et al. Perfusion CT: noninvasive surrogate marker for stratification of pancreatic cancer response to concurrent chemo- and radiation therapy. Radiology 2009;250:110-7. [Crossref] [PubMed]
- Jang KM, Kim SH, Min JH, et al. Value of diffusion-weighted MRI for differentiating malignant from benign intraductal papillary mucinous neoplasms of the pancreas. AJR Am J Roentgenol 2014;203:992-1000. [Crossref] [PubMed]
- Hanania AN, Bantis LE, Feng Z, et al. Quantitative imaging to evaluate malignant potential of IPMNs. Oncotarget 2016;7:85776-84. [Crossref] [PubMed]
- Cozzi L, Comito T, Fogliata A, et al. Computed tomography based radiomic signature as predictive of survival and local control after stereotactic body radiation therapy in pancreatic carcinoma. PLoS One 2019;14:e0210758. [Crossref] [PubMed]
- Chakraborty J, Midya A, Gazit L, et al. CT radiomics to predict high-risk intraductal papillary mucinous neoplasms of the pancreas. Med Phys 2018;45:5019-29. [Crossref] [PubMed]
- Permuth JB, Choi J, Balarunathan Y, et al. Combining radiomic features with a miRNA classifier may improve prediction of malignant pathology for pancreatic intraductal papillary mucinous neoplasms. Oncotarget 2016;7:85785-97. [Crossref] [PubMed]
- Wei R, Lin K, Yan W, et al. Computer-Aided Diagnosis of Pancreas Serous Cystic Neoplasms: A Radiomics Method on Preoperative MDCT Images. Technol Cancer Res Treat 2019;18:1533033818824339. [Crossref] [PubMed]
- Ren S, Zhao R, Zhang J, et al. Diagnostic accuracy of unenhanced CT texture analysis to differentiate mass-forming pancreatitis from pancreatic ductal adenocarcinoma. Abdom Radiol (NY) 2020;45:1524-33. [Crossref] [PubMed]
- Park S, Chu LC, Hruban RH, et al. Differentiating autoimmune pancreatitis from pancreatic ductal adenocarcinoma with CT radiomics features. Diagn Interv Imaging 2020;101:555-64. [Crossref] [PubMed]
- Ha J, Choi SH, Byun JH, et al. Meta-analysis of CT and MRI for differentiation of autoimmune pancreatitis from pancreatic adenocarcinoma. Eur Radiol 2021;31:3427-38. [Crossref] [PubMed]
- Wang Y, Hu X, Shi S, et al. Utility of Quantitative Metrics From Dual-Layer Spectral-Detector CT for Differentiation of Pancreatic Neuroendocrine Tumor and Neuroendocrine Carcinoma. AJR Am J Roentgenol 2022;218:999-1009. [Crossref] [PubMed]
- Ohki K, Igarashi T, Ashida H, et al. Usefulness of texture analysis for grading pancreatic neuroendocrine tumors on contrast-enhanced computed tomography and apparent diffusion coefficient maps. Jpn J Radiol 2021;39:66-75. [Crossref] [PubMed]
- Azoulay A, Cros J, Vullierme MP, et al. Morphological imaging and CT histogram analysis to differentiate pancreatic neuroendocrine tumor grade 3 from neuroendocrine carcinoma. Diagn Interv Imaging 2020;101:821-30. [Crossref] [PubMed]
- Noh SH, Park SR, Yang HK, et al. Adjuvant capecitabine plus oxaliplatin for gastric cancer after D2 gastrectomy (CLASSIC): 5-year follow-up of an open-label, randomised phase 3 trial. Lancet Oncol 2014;15:1389-96. [Crossref] [PubMed]
- Jiang Y, Li T, Liang X, et al. Association of Adjuvant Chemotherapy With Survival in Patients With Stage II or III Gastric Cancer. JAMA Surg 2017;152:e171087. [Crossref] [PubMed]
- Razzak M. Genetics: new molecular classification of gastric adenocarcinoma proposed by The Cancer Genome Atlas. Nat Rev Clin Oncol 2014;11:499. [Crossref] [PubMed]
- Jiang Y, Yuan Q, Lv W, et al. Radiomic signature of (18)F fluorodeoxyglucose PET/CT for prediction of gastric cancer survival and chemotherapeutic benefits. Theranostics 2018;8:5915-28. [Crossref] [PubMed]
- Martini I, Polici M, Zerunian M, et al. CT texture analysis of liver metastases in PNETs versus NPNETs: Correlation with histopathological findings. Eur J Radiol 2020;124:108812. [Crossref] [PubMed]
- Li W, Zhang L, Tian C, et al. Prognostic value of computed tomography radiomics features in patients with gastric cancer following curative resection. Eur Radiol 2019;29:3079-89. [Crossref] [PubMed]
- Hou Z, Ren W, Li S, et al. Radiomic analysis in contrast-enhanced CT: predict treatment response to chemoradiotherapy in esophageal carcinoma. Oncotarget 2017;8:104444-54. [Crossref] [PubMed]
- Sah BR, Owczarczyk K, Siddique M, et al. Radiomics in esophageal and gastric cancer. Abdom Radiol (NY) 2019;44:2048-58. [Crossref] [PubMed]
- Nishida T, Blay JY, Hirota S, et al. The standard diagnosis, treatment, and follow-up of gastrointestinal stromal tumors based on guidelines. Gastric Cancer 2016;19:3-14. [Crossref] [PubMed]
- Min KW, Leabu M. Interstitial cells of Cajal (ICC) and gastrointestinal stromal tumor (GIST): facts, speculations, and myths. J Cell Mol Med 2006;10:995-1013. [Crossref] [PubMed]
- Shah R, Jonnalagadda S. The GIST of a stromal tumor. Gastroenterology 2005;128:2170-1; discussion 2171-2. [Crossref] [PubMed]
- Crocetti D, Sapienza P, Cisano C, et al. Pancreas preserving surgery for duodenal gastrointestinal stromal tumor removal. Minerva Chir 2016;71:281-5. [PubMed]
- Chen T, Ning Z, Xu L, et al. Radiomics nomogram for predicting the malignant potential of gastrointestinal stromal tumours preoperatively. Eur Radiol 2019;29:1074-82. [Crossref] [PubMed]
- Wang C, Li H, Jiaerken Y, et al. Building CT Radiomics-Based Models for Preoperatively Predicting Malignant Potential and Mitotic Count of Gastrointestinal Stromal Tumors. Transl Oncol 2019;12:1229-36. [Crossref] [PubMed]
- Ning Z, Luo J, Li Y, et al. Pattern Classification for Gastrointestinal Stromal Tumors by Integration of Radiomics and Deep Convolutional Features. IEEE J Biomed Health Inform 2019;23:1181-91. [Crossref] [PubMed]
- Kiryu S, Akai H, Nojima M, et al. Impact of hepatocellular carcinoma heterogeneity on computed tomography as a prognostic indicator. Sci Rep 2017;7:12689. [Crossref] [PubMed]
- Zhou Y, He L, Huang Y, et al. CT-based radiomics signature: a potential biomarker for preoperative prediction of early recurrence in hepatocellular carcinoma. Abdom Radiol (NY) 2017;42:1695-704. [Crossref] [PubMed]
- Hu HT, Wang Z, Huang XW, et al. Ultrasound-based radiomics score: a potential biomarker for the prediction of microvascular invasion in hepatocellular carcinoma. Eur Radiol 2019;29:2890-901. [Crossref] [PubMed]
- Zhou W, Zhang L, Wang K, et al. Malignancy characterization of hepatocellular carcinomas based on texture analysis of contrast-enhanced MR images. J Magn Reson Imaging 2017;45:1476-84. [Crossref] [PubMed]
- West DL, Kotrotsou A, Niekamp AS, et al. CT-based radiomic analysis of hepatocellular carcinoma patients to predict key genomic information. J Clin Oncol 2017;35:e15623. [Crossref]
- Bridgewater J, Galle PR, Khan SA, et al. Guidelines for the diagnosis and management of intrahepatic cholangiocarcinoma. J Hepatol 2014;60:1268-89. [Crossref] [PubMed]
- Chu H, Liu Z, Liang W, et al. Radiomics using CT images for preoperative prediction of futile resection in intrahepatic cholangiocarcinoma. Eur Radiol 2021;31:2368-76. [Crossref] [PubMed]
- Ji GW, Zhu FP, Zhang YD, et al. A radiomics approach to predict lymph node metastasis and clinical outcome of intrahepatic cholangiocarcinoma. Eur Radiol 2019;29:3725-35. [Crossref] [PubMed]
- Hu J, Chen FY, Zhou KQ, et al. Intrahepatic cholangiocarcinoma patients without indications of lymph node metastasis not benefit from lymph node dissection. Oncotarget 2017;8:113817-27. [Crossref] [PubMed]
- Chen Y, Liu H, Zhang J, et al. Prognostic value and predication model of microvascular invasion in patients with intrahepatic cholangiocarcinoma: a multicenter study from China. BMC Cancer 2021;21:1299. [Crossref] [PubMed]
- Zhou Y, Zhou G, Zhang J, et al. Radiomics signature on dynamic contrast-enhanced MR images: a potential imaging biomarker for prediction of microvascular invasion in mass-forming intrahepatic cholangiocarcinoma. Eur Radiol 2021;31:6846-55. [Crossref] [PubMed]
- Aujay G, Etchegaray C, Blanc JF, et al. Comparison of MRI-based response criteria and radiomics for the prediction of early response to transarterial radioembolization in patients with hepatocellular carcinoma. Diagn Interv Imaging 2022;103:360-6. [Crossref] [PubMed]
- Mosconi C, Cucchetti A, Bruno A, et al. Radiomics of cholangiocarcinoma on pretreatment CT can identify patients who would best respond to radioembolisation. Eur Radiol 2020;30:4534-44. [Crossref] [PubMed]
- Liu X, Khalvati F, Namdar K, et al. Can machine learning radiomics provide pre-operative differentiation of combined hepatocellular cholangiocarcinoma from hepatocellular carcinoma and cholangiocarcinoma to inform optimal treatment planning? Eur Radiol 2021;31:244-55. [Crossref] [PubMed]
- Lewis S, Peti S, Hectors SJ, et al. Volumetric quantitative histogram analysis using diffusion-weighted magnetic resonance imaging to differentiate HCC from other primary liver cancers. Abdom Radiol (NY) 2019;44:912-22. [Crossref] [PubMed]
- Kim KH, Lee SG, Park EH, et al. Surgical treatments and prognoses of patients with combined hepatocellular carcinoma and cholangiocarcinoma. Ann Surg Oncol 2009;16:623-9. [Crossref] [PubMed]
- Tsochatzis EA, Bosch J, Burroughs AK. Liver cirrhosis. Lancet 2014;383:1749-61. [Crossref] [PubMed]
- Altamirano-Barrera A, Barranco-Fragoso B, Méndez-Sánchez N. Management strategies for liver fibrosis. Ann Hepatol 2017;16:48-56. [Crossref] [PubMed]
- Aguirre DA, Behling CA, Alpert E, et al. Liver fibrosis: noninvasive diagnosis with double contrast material-enhanced MR imaging. Radiology 2006;239:425-37. [Crossref] [PubMed]
- Venkatesh SK, Yin M, Takahashi N, et al. Non-invasive detection of liver fibrosis: MR imaging features vs. MR elastography. Abdom Imaging 2015;40:766-75. [Crossref] [PubMed]
- Park HJ, Lee SS, Park B, et al. Radiomics Analysis of Gadoxetic Acid-enhanced MRI for Staging Liver Fibrosis. Radiology 2019;290:380-7. [Crossref] [PubMed]
- Naganawa S, Enooku K, Tateishi R, et al. Imaging prediction of nonalcoholic steatohepatitis using computed tomography texture analysis. Eur Radiol 2018;28:3050-8. [Crossref] [PubMed]
- Samir AE, Dhyani M, Vij A, et al. Shear-wave elastography for the estimation of liver fibrosis in chronic liver disease: determining accuracy and ideal site for measurement. Radiology 2015;274:888-96. [Crossref] [PubMed]
- Zheng J, Guo H, Zeng J, et al. Two-dimensional shear-wave elastography and conventional US: the optimal evaluation of liver fibrosis and cirrhosis. Radiology 2015;275:290-300. [Crossref] [PubMed]
- Wang K, Lu X, Zhou H, et al. Deep learning Radiomics of shear wave elastography significantly improved diagnostic performance for assessing liver fibrosis in chronic hepatitis B: a prospective multicentre study. Gut 2019;68:729-41. [Crossref] [PubMed]
- Rimola J, Rodriguez S, García-Bosch O, et al. Magnetic resonance for assessment of disease activity and severity in ileocolonic Crohn's disease. Gut 2009;58:1113-20. [Crossref] [PubMed]
- Miles KA, Ganeshan B, Hayball MP. CT texture analysis using the filtration-histogram method: what do the measurements mean? Cancer Imaging 2013;13:400-6. [Crossref] [PubMed]
- Makanyanga J, Ganeshan B, Rodriguez-Justo M, et al. MRI texture analysis (MRTA) of T2-weighted images in Crohn's disease may provide information on histological and MRI disease activity in patients undergoing ileal resection. Eur Radiol 2017;27:589-97. [Crossref] [PubMed]
- Bhatnagar G, Makanyanga J, Ganeshan B, et al. MRI texture analysis parameters of contrast-enhanced T1-weighted images of Crohn's disease differ according to the presence or absence of histological markers of hypoxia and angiogenesis. Abdom Radiol (NY) 2016;41:1261-9. [Crossref] [PubMed]
- Song J, Yin Y, Wang H, et al. A review of original articles published in the emerging field of radiomics. Eur J Radiol 2020;127:108991. [Crossref] [PubMed]
- Berenguer R, Pastor-Juan MDR, Canales-Vázquez J, et al. Radiomics of CT features may be nonreproducible and redundant: influence of CT acquisition parameters. Radiology 2018;288:407-15. [Crossref] [PubMed]
- Meyer M, Ronald J, Vernuccio F, et al. Reproducibility of CT radiomic features within the same patient: influence of radiation dose and CT reconstruction settings. Radiology 2019;293:583-91. [Crossref] [PubMed]
Cite this article as: De Santis D, Del Gaudio A, Zerunian M, Polici M, Guido G, Tarallo M, Masci B, Ubaldi N, Iannicelli E, Laghi A, Caruso D. Radiomics analysis in gastrointestinal imaging: a narrative review. Dig Med Res 2023;6:17.