CDK1/TTR/MYC promote the formation of metastatic niches and affect the prognosis of pancreatic cancer by participating in immune CD4+ T cell infiltration as indicated by an integrated bioinformatics analysis
Introduction
Pancreatic cancer is a disease with a poor outcome, characterized by a low survival rate and an extremely high mortality rate that is similar to the morbidity rate. According to the GLOBOCAN 2012 estimates, 331,000 people die from pancreatic cancer each year, and it is ranked as the 7th leading cause of cancer death (1). Because the pancreas is located in the abdominal area in front of the spine and behind the stomach, it is difficult to determine and diagnose in early pancreatic cancer. It is not apparent that the symptoms, including jaundice, pain, loss of appetite, pancreatitis and weight loss, in the initial stage of pancreatic cancer, are indicative of pancreatic cancer which is thus not easy to detect (2). Metastasis to the liver, peritoneum, lymph nodes, lung, fat, and diaphragm is the critical reason for the extremely low 5-year survival rate for pancreatic cancer patients.
Metastasis, as the direct causal factor of cancer-related fatal outcome, is a dynamic and complex multidimensional process. It is related not only to genetic mutations in cancer cells, but also to the tumor microenvironment (3). In 1889, Paget et al. give an account of the “seed-soil” theory, explaining that tumor metastasis relies on the interaction between cancer cells (seed) and the host microenvironment (soil) (4). In 1928, James Ewing held a different opinions, explaining that several lines of evidence suggest a potential role of hemodynamics in tumor metastasis and spread (5). In the 1970s, Isaiah Fidler’s research definitely indicated that metastasis is organ-specific (6). From the perspective of the tumor itself, tumor cells induce the formation of the environment in remote organs to promote the survival and development of cancer cells. The specific microenvironment is defined as pre-metastasis niche (PMN). Blood coagulation and vascular destruction are believed to be the initial events of PMN formation. Subsequently, a series of changes occurring in native tissue of distant organs are favorable for the formation of metastatic niches. An example of this fact is the possibility that cytokines, such as MMP, contribute to vascular disorders, promote extracellular matrix (ECM) degradation, and facilitate the infiltration of circulating tumor cells (CTCs) through autocrine or paracrine modes of action. Adhesion molecules carried by tumor-derived exosomes, such as integrins, can bind to ECM molecules in the PMN of specific organs, which determines organ-specific transfer, and attracting bone marrow-derived cells (BMDCs) through recruitment, native cells attract CTCs to adhere to endothelial cells, which overglow and form metastatic niches. According to an analysis, external factors, such as surgery, infection, age and other factors, can affect the formation of the premetastatic niche (PMN) and metastatic niche (7).
However, the data on the pre-metastatic and metastatic niches of pancreatic cancer are less clear due to technical limitations, such as the lack of specific probes to track PMNs and the difficulty of obtaining tissues prior to metastasis. In the current study we explore the hypothetical mechanism of the formation of pancreatic cancer pre-metastatic and metastatic niches by means of analyzing the pancreatic cancer metastasis data set from the GEO database. The differentially expressed genes (DGs) that affect the formation of metastatic niches and the prognosis of pancreatic cancer were identified. Potential therapeutic targets for pancreatic cancer were found by immune cell infiltration and survival analysis, which provided new ideas for clinical molecular targeted therapy and immunotherapy.
We present the following article in accordance with the MDAR checklist (available at http://dx.doi.org/10.21037/dmr-20-98).
Methods
The study was conducted in accordance with the Declaration of Helsinki (as revised in 2013).
Gene expression data sets
All of the data used in this research are in the public domain. The GSE71729 microarray data set was screened from the Gene Expression Omnibus (GEO) database by two keywords, “pancreatic cancer” and “metastasis”. The search data yielded 145 primary and 61 metastatic PDAC tumors, 17 cell lines, 446 pancreas and 88 distant sites with adjacent normal samples. Then click to “Define group” and divide the data into 4 groups, including normal lymph node group, lymph node metastasis in pancreatic cancer group, non-metastasis in pancreatic cancer group and normal pancreas group, according to whether there is lymph node metastasis. After clicking “GEO2R” and “Analyze”, the DGs would be automatically generated. The same principle can be used to screen out the corresponding number of DGs according to the presence or absence of liver, diaphragm, fat, lung, peritoneal metastasis. To guarantee the reliability of the study, 10 fixed samples in the pancreatic cancer non-metastatic group and normal pancreas group were selected. The GEO2R analysis data were evaluated using median values, and a P value less than 0.05 was considered significant. The specific groupings and the number of cases are shown in Table 1.
Table 1
Metastasis niches | Groups | Number of cases | Number of different genes |
---|---|---|---|
Lymph node metastasis | Normal lymph node group | 10 | 7,538 |
Lymph node metastasis in pancreatic cancer group | 9 | ||
Non-metastasis in pancreatic cancer group | 10 | ||
Normal pancreas group | 10 | ||
Liver metastasis | Normal liver group | 25 | 11,455 |
Liver metastasis in pancreatic cancer group | 25 | ||
Non-metastasis in pancreatic cancer group | 10 | ||
Normal pancreas group | 10 | ||
Diaphragm metastasis | Normal diaphragm group | 10 | 8,813 |
Diaphragm metastasis in pancreatic cancer group | 3 | ||
Non-metastasis in pancreatic cancer group | 10 | ||
Normal pancreas group | 10 | ||
Fat metastasis | Normal fat | 6 | 3,589 |
Fat metastasis in pancreatic cancer group | 2 | ||
Non-metastasis in pancreatic cancer group | 10 | ||
Normal pancreas group | 10 | ||
Lung metastasis | Normal liver group | 19 | 6,633 |
Lung metastasis in pancreatic cancer group | 8 | ||
Non-metastasis in pancreatic cancer group | 10 | ||
Normal pancreas group | 10 | ||
Peritoneal metastasis | Normal liver group | 3 | 4,319 |
Peritoneal metastasis in pancreatic cancer group | 7 | ||
Non-metastasis in pancreatic cancer group | 10 | ||
Normal pancreas group | 10 |
GSE71729 data set was found after entering the keywords “pancreatic cancer” and “metastasis” in the GEO database, then click to “Define group” and divide the data into 4 groups, including normal lymph node group, lymph node metastasis in pancreatic cancer group, non-metastasis in pancreatic cancer group and normal pancreas group, according to whether there is lymph node metastasis. After clicking “GEO2R” and “Analyze”, the differential genes will be automatically generated. The fourth column shows the number of DGs that have been screened out. The same principle can be used to screen out the corresponding number of DGs according to the presence or absence of liver, diaphragm, fat, lung, peritoneal metastasis.
Venn diagram
To screen out the coexpressed DGs in various metastatic niches of pancreatic cancer, a Venn diagram was generated by FunRich (http://funrich.org/index.html) (8). First, these DGs were introduced in the system, and a “Venn Diagram” was generated. Furthermore, the outcome, as shown in Figure 1, was determined by selecting the required subset. The results of the Venn diagramming with the four subsets, including lymph node metastasis niches, peritoneal metastasis niches, lung metastasis niches and liver metastasis niches, are shown in Figure 1A, then the DGs coexpressed by these first four groups were analyzed with DGs in the fat metastasis niches and diaphragm metastases. The analysis results of all transfer niches showing in Figure 1B,C.
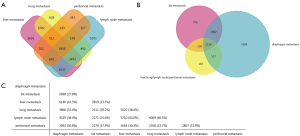
Gene ontology (GO) enrichment analysis
The aim is to thoroughly explore the characteristics common to different metastatic niches of pancreatic cancer. The different genes coexpressed by six various metastatic niches of pancreatic cancer in the Venn diagram were entered into FunRich as a subset. Then, a GO enrichment analysis was performed from multiple perspectives, biological pathways, biological process (BP), cell component (CC), clinical phenotype (CP), COSMIC, molecular function (MF), protein domain, site of expression, and transcription factor, were categorized using FunRich. The screening criterion was a P values less than 0.05. The histogram results are presented in Figure 2.
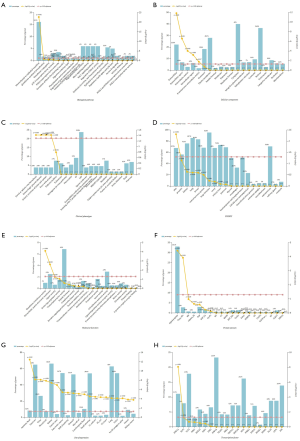
Protein-protein interaction (PPI) network
The probable correlation between different co-expressed genes was analyzed using the online tools STRING (http://string-db.org/, Figure 3) (9). A relationship with a combined score >0.4 was selected as the node of the PPI network. The score is commensurately associated with the protein relationship, the higher the combined score, the closer the relationship between the two proteins in the network. Subsequently, these relationship node score data, obtained from the network diagram using STRING, were derived and used for CytoHubba by setting the parameters—for Nodes’ score, Calculate, and algorithm selection degree. According to the Degree algorithm, the gene function ranking was predicted, and the top 20 pivotal genes were selected as hub genes (Figure 4 and Table 2). The higher the score, the darker the color of the label, indicating that it has a closer relationship with other proteins.
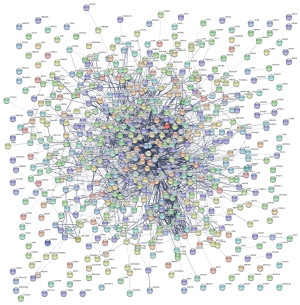
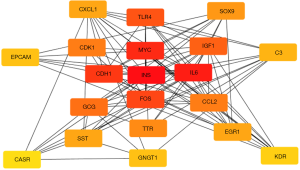
Table 2
Rank | Gene | Score |
---|---|---|
1 | INS | 111 |
2 | IL6 | 88 |
3 | MYC | 75 |
4 | CDH1 | 66 |
5 | FOS | 56 |
6 | TLR4 | 48 |
7 | IGF1 | 45 |
8 | GCG | 43 |
9 | CDK1 | 34 |
9 | CCL2 | 34 |
11 | TTR | 32 |
12 | SOX9 | 31 |
13 | EGR1 | 30 |
13 | CXCL1 | 30 |
13 | SST | 30 |
16 | C3 | 29 |
17 | EPCAM | 28 |
17 | GNGT1 | 28 |
19 | KDR | 27 |
20 | CASR | 26 |
It reflects the close relationship between protein and protein in network diagram using CytoHabble. A higher score indicates a closer relationship between protein and protein. Among them, INS, IL-6 and MYC have the highest relationship node scores, and the gene function ranking is the highest.
Heat map
Twenty critical metastatic-related genes, which were screened out by CytoHubba, were introduced in TCGA analysis using UALCAN (10). The TCGA dataset is restricted to pancreatic adenocarcinoma. Subsequently, click “Explore” and “Heatmap for query genes in turn”. See Figure 5 for details.
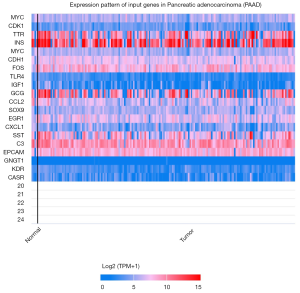
Survival analysis
In this study, two methods of survival analysis were adopted to reduce errors caused by differences in the platforms. First, the cancer type analysis in the GEPIA (11) was exploited to calculate the overall survival rate of the highest 20 hub genes based on gene name and pancreatic adenocarcinoma (PAAD). Kaplan-Meier survival curves were plotted with P values of 0.05 representing statistical significance. Second, the survival analysis function of the TIMER (12) platform was used to obtain the survival curve of the genes in pancreatic cancer, a P value less than 0.05 was the screening condition. Subsequently, the relationship between diverse immune infiltrating cells and the prognosis of pancreatic cancer was also discovered in TIMER.
Immune cell infiltration analysis
In this study, we used the TIMER platform to further explore the probable mechanism of metastasis related hub genes affecting pancreatic cancer metastasis and prognosis. Once metastatic related hub gene names and PAAD were entered into the TIMER gene analysis system, the Cox regression results, including hazard ratios and statistical significance, were determined. Similarly, Kaplan-Meier plots for immune infiltrates and genes, used to visualize the survival differences were loaded. A P<0.05 for log-rank test used to compare the survival curves indicated that gene expression is a direct result of specific immune cell infiltration, such as B cells, CD4+ T cells, CD8+ T cells, macrophages, neutrophils, and dendritic cells. Similarly, box charts were generated from the SCNA analysis of TIMER, and they presented the comparison of tumor somatic mutation copy number and tumor immune infiltration level. Among these results, the different intervals of the P value are defined as follows: 0≤***<0.001≤**<0.01≤*<0.05.
Statistical analysis
Median was used for GEO2R analysis. Regression algorithm was used to screen 20 key genes through cytoHubba. Kaplan-Meier plots were used for survival analysis and immune infiltration. All tests were p values less than 0.05 were considered statistically significant.
Results
DG screening base on various metastatic niches in pancreatic cancer
As explained above, the data set GSE71729 for pancreatic cancer provides information on different metastatic niches. The screening results of the GEO2R analysis are exhibited in Table 1. The four column of Table 1 represents the number of DGs screened out. A total of 7,538 DGs were identified in lymph node metastatic niches. In addition, 11,455, 8,813, 3,589, 6,633 and 4,319 DGs were recognized in liver, diaphragm, fat, lung and peritoneal metastasis niches, respectively.
Identification of coexpressed DGs in various metastatic niches
The abovementioned DGs of various metastatic niches were placed in a Venn diagram, and the coexpressed DGs were filtered. The results are shown in Figure 1. A total of 1,956 DGs were identified and coexpressed in the metastatic niches of pancreatic cancer with lymph node, liver, lung and peritoneum at the intersection of the Venn diagram (Figure 1A). A total of 1,116 DGs co-expressed in six metastatic niches of pancreatic cancer including lymph nodes, liver, lungs, peritoneum, diaphragm, and fat were found at the intersection of the Venn diagram, and are shown in Figure1B,C.
Functional enrichment analysis
The functional enrichment analysis of 1,116 DGs coexpressed in various metastatic niches was performed using FunRich with the intention of further exploring the mechanism of metastatic niches formation in pancreatic cancer, and a total of five results were obtained: First, in terms of signaling pathways, approximately 16.1% of the DGs exert biological effects associated with the epithelial-to-mesenchymal transition (EMT) (P<0.001) pathway (Figure 2A). No other shared signaling pathways were discovered, and there were no valuable discoveries in the BPs category. Second, from the perspective of cell composition, almost one-quarter of the DGs had extracellular and extracellular matrices (P<0.001) (Figure 2B). Third, in terms of clinical manifestations, various clinical symptoms, including portal or splenic vein thrombosis, hemorrhagic pleural effusion, urinary excretion of lysine and cystine, and fever with attacks, suggest the existence of metastatic niches (Figure 2C). Fourth, from the somatic mutation analysis, 67.9% of DGs are associated with somatic skin mutations (Figure 2D). Fifth, from the perspective of MF, 1% of the DGs have glutathione transferase activity (P<0.001), and 2.5% of the DGs participate as ECM structural constituents (P<0.001) (Figure 2E). Sixth, from the aspect of the protein domain, 33.1% of the DGs participate in the formation of signal peptides, and 2.8% of the DGs have Tryp_SPc fragments (Figure 2F). Seventh, the DGs are a widely expressed: amniotic fluid, pancreas, urine, MicroProt2, kidney, gastric juice, pancreatic cancer, BOB_Sensitivity, stomach, MicroProt, cerebrospinal fluid, small intestine, 21_unfract, 20_cys, 22_mars, lung, and colon, b1-SE_ninglyco_qstar, semen, kidney cancer (Figure 2G). Eighth, 11.1% of the DGs are closely related to the transcription factor HNF1A (Figure 2H).
Metastasis-related PPIs and identification of the top 20 hub genes in pancreatic cancer
The PPI diagram was generated by STRING is shown in Figure 3. Subsequently, top 20 most valuable DGs were identified using the cytoHubba platform, and the specific relationship scores is exhibited in Table 2. The results indicated that the top 20 pivotal genes form a complex relationship network centered on MYC with the key nodes such as insulin (INS), interleukin 6 (IL6), cadherin 1 (CDH1), Fos proto-oncogene (FOS), Toll like receptor 4 (TLR4), insulin like growth factor 1 (IGF1), glucagon (GCG), cyclin-dependent kinase 1 (CDK1), C-C motif chemokine ligand 2 (CCL2), transthyretin (TTR), SRY-box transcription factor 9 (SOX9), early growth response 1 (EGR1), C-X-C motif chemokine ligand 1 (CXCL1), somatostatin (SST), complement C3 (C3), epithelial cell adhesion molecule (EPCAM), G protein subunit gamma transducin 1 (GNGT1) and kinase insert domain receptor (KDR) (Figure 4), and heat map results of 20 top genes using UALCAN were shown in Figure 5.
Identification of genes related to pancreatic cancer metastasis and prognosis
The GEPIA survival analysis results (Figure 6A) showed that the P values of the survival curve of only three genes among the top 20 critical pancreatic cancer metastasis-related genes, namely, MYC (P=0.0085), CDK1 (P=0.0006) and TTR (P=0.011), were statistically significant. An additional 16 pivotal genes were exceeded because the P values of the survival curve ≥0.05 (Table 3). To enhance the reliability of the study, a survival analysis using TIMER was added to this experiment. The consequences of using two platforms were the same in those shown in the survival curve. The P values of MYC, CDK1 and TTR were statistically significant (Figure 6B). The difference, however, was that the P value of the survival curves of IL-6 and CCL2 were dissimilar in the two platforms, therefore, they were excluded from this experiment. Therefore, MYC, CDK1 and TTR were selected as hub genes for pancreatic cancer metastasis and prognosis, and the other 17 pivot genes were related only to pancreatic cancer metastasis, but not directly related to the prognosis of pancreatic cancer (Table 3).
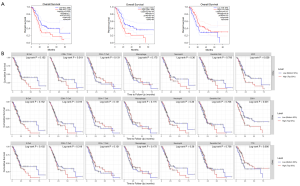
Table 3
Log-rank P value of survival analysis | GEPIA | TIMER |
---|---|---|
INS | 0.74 | 0.691 |
IL6 | 0.048 | 0.055 |
CDH1 | 0.25 | 0.117 |
FOS | 0.52 | 0.17 |
TLR4 | 0.41 | 0.504 |
IGF1 | 0.65 | 0.992 |
GCG | 0.22 | 0.157 |
CCL2 | 0.064 | 0.045 |
SOX9 | 0.33 | 0.358 |
EGR1 | 0.26 | 0.464 |
CXCL1 | 0.67 | 0.714 |
SST | 0.16 | 0.092 |
C3 | 0.52 | 0.805 |
EPCAM | 0.26 | 0.764 |
GNGT1 | 0.8 | 0.921 |
KDR | 0.22 | 0.331 |
CASR | 0.97 | 0.791 |
The P value of most gene survival curves is greater than 0.5, which is not statistically significant, and these genes are not directly related to the prognosis of pancreatic cancer. Among them, the survival curves of IL6 and CCL2 were different, so they were excluded.
Correlation between immune infiltration and pancreatic cancer metastasis and prognosis
The TIMER survival analysis results showed that the infiltration of various immune cells, including B cells, CD8+ T cells, CD4+ T cells, macrophages, neutrophils, and dendritic cells, was not directly correlated with the prognosis of pancreatic cancer (Figure 6B).
Three genetic analysis results were obtained from the TIMER analysis (Figure 7A). First, MYC gene expression was exactly correlated with the infiltration of various immune cells including B cells, CD8+ T cells, CD4+ T cells, macrophages, neutrophils, and dendritic cells, and their P values were statistically significant. Second, CDK1 gene expression has a true direct correction with immune infiltration of CD4+ T cells, and had no relationship to the infiltration of the other immune cells. Finally, the expression of the TTR gene was directly related to CD4+ T cells and macrophages, both were statistically significant at the P<0.05 level, and it had no direct correction with the other infiltrating immune cells. Moreover, there was a correlation between the expression of CDK1 and the TTR gene, with statistical significance at the P<0.05 level, while MYC had no direct correlation with CDK1 and TTR, with a P>0.05, any association was not statistically significant.
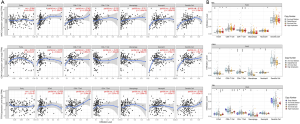
The comparison result of immune cell infiltration levels among pancreatic cancer with different somatic copy number alterations in MYC, CDK1 or TTR were shown in Figure 7B.
Discussion
Pancreatic cancer is well-known for its low survival rate and high mortality rate. Experts speculate that by 2025, pancreatic cancer will become the second leading cause of cancer death, and its survival rate will be not more than 10% (13). The high risk of metastasis is a critical cause of death from pancreatic cancer. Thus the study of the initial events of metastasis appears particularly important. Before a cancer has metastasized, a series of changes in potential conversion sites, such as liver and lung, may occur, making them more conducive to cancer cell implantation and growth. This changed local microenvironment is defined as a PMNs (14). However, there are limited studies on the formation mechanism of pancreatic cancer pre-metastasis niches.
In this study, we performed a series of analyses on various metastatic niches, including liver, lung, lymph nodes, peritoneum, diaphragm and fat, using the pancreatic cancer GSE71729 dataset. It was found that 1,116 DGs were coexpressed in 6 different metastatic niches. The GO results showed that these genes are extensively expressed in gastrointestinal pancreas, lung, kidney, urine, gastric fluid, cerebrospinal fluid, amniotic fluid, other body fluids, etc. Exosomes, substances abundant in body fluids, play important roles in tumor metastasis and drug resistance (15); therefore, it is speculated that these metastasis-related genes can regulate tumor metastasis through exosomes. Furthermore, these genes are associated with multiple common clinical manifestations, including portal or splenic vein thrombosis, hemorrhagic pleural effusion, urinary excretion of lysine and cystine, and fever, suggesting the possibility of tumor metastases, typically referred to as metastatic symptoms. A total of 5.1% of pancreatic cancer metastasis-related genes have glutathione S-transferase (GST) activity, as indicated in the gene enrichment analysis. The GST group comprises enzymes involved in liver detoxification; cell signal transduction; and anti-apoptosis, inflammatory and pro-inflammatory reactions. GST alpha 1 (GSTA1) is mainly present in the liver, kidney and testis; GST Pi 1 (GSTP1) is highly expressed in extrahepatic tissues; and GST theta 1 (GSTT1) is mainly expressed in the kidney and liver. The GST genotype affects the occurrence and evolution of cancer. GST polymorphism affects cancer sensitivity, and GSTP1 epigenetic silencing occurs early in cancer (16). The inflammatory response in the tumor metastatic microenvironment inseparable related to the GST activity of metastatic-related genes. A total of 11.1% of the pancreatic cancer metastasis-related genes were cross referenced to hepatic nuclear factor (HNF1α), a transcription factor whose encoded protein is expressed in liver specific genes. Therefore, it is speculated that the formation of liver metastases in niches probably has some connection with the GST activity of metastatic-related genes. HNF1α genetic defects are causes of certain diseases, including maturity-onset diabetes of the young, type 3 insulin-dependent diabetes mellitus, and hepatic adenoma. It is also engaged in the development and heterogeneity of the interleukin (ILC) family and the regulation pathways of islet beta-cell development. Recent studies have shown that HNF1α is a novel oncogene involved in regulating the characteristics of pancreatic cancer stem cells (17). However, little research has been done to focus on the role of HNF1α in pancreatic cancer metastasis.
Abundant tumor stroma (more than 50%) is the most obvious feature of the tumor microenvironment of pancreatic cancer. Degradation of the extracellular matrix, the main component of the tumor stroma, is a prerequisite for tumor metastasis (18). The current study found that 2.5% of pancreatic cancer metastasis-related genes are involved with the ECM structure, indicating that some metastasis-related genes participate in the ECM degradation process and thus prepare for cancer metastasis before the tumor metastasis occurs. A total of 16.1% of pancreatic cancer metastasis-related genes participate in the EMT, through which tumor cells acquire a metastatic phenotype that is characterized by the downregulation of cadherin, cytoskeletal reorganization, and enhanced migration and invasion capabilities (19). Simultaneously, three types of EMT are described: type I occurs in the tissues and organs formed by gastrointestinal embryos during embryo development, type II occurs in the process of adult fibrosis caused by tissue damage or inflammation, and type III is closely related to cancer metastasis. Calreticulin promotes pancreatic cancer cell EMT through the integrin/epidermal growth factor receptor (EGFR)-extracellular signal-regulated kinase (ERK)/mitogen-activated protein kinase (MAPK) signaling pathway (20). As a positive signal of tumor metastasis, the EMT plays a vital role in the formation of tumor metastases.
In other refractory cancers, immunotherapy has led to an unprecedented remission. However, there is little research on the application of immunotherapy to pancreatic cancer. The current study discovered that the majority of 20 critical genes related to pancreatic cancer metastasis are involved in immune cell infiltration. Among these, only three genes are related to the metastasis and prognosis of pancreatic cancer: MYC, CDK1, and TTR. The gene expression of MYC is a direct result of the infiltration of various immune cells including B cells, CD4+ T cells, CD8+ T cells, macrophages, neutrophils and dendritic cells. TTR gene expression is a direct result of macrophage and CD4+ T cell immune infiltration, which is related to the expression of CDK1. There is a correlation between CDK1 and TTR gene expression, and both are linked to CD4+ T cell immune infiltration. Although MYC is not relevant to the gene expression of CDK1 and TTR, it is directly linked to CD4+ T cell immune infiltration. In summary, MYC, CDK1 and MYC can promote the formation of pancreatic cancer metastasis niches by regulating the activity of CD4+ T cells to affect the prognosis of pancreatic cancer. CDK1 is a gene encoding a serine/tyrosine protein kinase, which is associated with G1/S or G2/M cell cycle transition in pancreatic cancer (21). It is expressed in prostate cancer (22,23), colorectal cancer (24), and lymphoma (25), and is linked to poor prognosis in breast cancer (26) and ovarian epithelial cancer (27,28). CDK1 has an obvious anticancer effect (29), and promotes the infiltration of malignant melanoma cells as facilitated by SRY-box transcription factor 2 (SOX2) (30). The TTR gene encodes the thyroxine transporter, one of three pre-albumins, and it is associated with the transport of thyroid hormone in plasma and cerebrospinal fluid, proteolysis, nerve regeneration, autophagy and glucose homeostasis. Mutation to this gene is connected to amyloid deposition, and the overexpression of the encoded protein is related to prostate cancer metastasis (31) and gastric cancer prognosis (32), in which it promotes tumor growth by regulating tumor immunity and endothelial cells (33). In the clinic, simultaneous testing of CA199 and TTR can be used for the early detection of pancreatic cancer (34). MYC, a proto-oncogene encoding nuclear phosphor-protein, plays a significant role in cell cycle progression, apoptosis, and transformation. Numerous diseases including Burkitt’s lymphoma, high-grade B cell lymphoma and multiple myeloma are associated with the gene translocation of MYC. Sancho et al. have shown that MYC/PGC-1α (peroxisome proliferator-activated receptor-γ coactivator 1α) determines the metabolic phenotype and plasticity of pancreatic cancer stem cells (35).
In this study, the formation of pancreatic cancer metastatic niches was found to involve a combination of multiple signaling pathways, including those associated with the EMT, ECM remodeling, immune cell infiltration, the acquisition of stem cell characteristics, and angiogenesis. MYC/CDK1/TTR, are key genes in the formation of the metastatic niche, directly impacting the prognosis of pancreatic cancer (Figure 8). All three are expected to become potential targets for immunotherapy and molecular therapy in pancreatic cancer. The focus of subsequent experiments is to further verify the relationship between MYC, CDK1, and TTR in vivo and in vitro with the metastasis and prognosis of pancreatic cancer, as well as the correlation with CD4 immune infiltration.
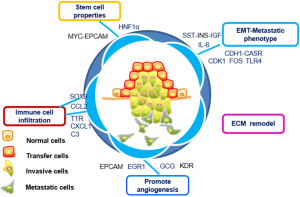
Acknowledgments
This work was supported by multiple public platforms including GEO, FunRich, ULCAN, TIMER, Cytoscape, and GEPIA.
Funding: None.
Footnote
Reporting Checklist: The authors have completed the MDAR checklist. Available at http://dx.doi.org/10.21037/dmr-20-98
Conflicts of Interest: All authors have completed the ICMJE uniform disclosure form (available at http://dx.doi.org/10.21037/dmr-20-98). The authors have no conflicts of interest to declare.
Ethical Statement: The authors are accountable for all aspects of the work in ensuring that questions related to the accuracy or integrity of any part of the work are appropriately investigated and resolved. The study was conducted in accordance with the Declaration of Helsinki (as revised in 2013). The data in this study are all from online public databases, individual consent for this retrospective analysis was waived.
Open Access Statement: This is an Open Access article distributed in accordance with the Creative Commons Attribution-NonCommercial-NoDerivs 4.0 International License (CC BY-NC-ND 4.0), which permits the non-commercial replication and distribution of the article with the strict proviso that no changes or edits are made and the original work is properly cited (including links to both the formal publication through the relevant DOI and the license). See: https://creativecommons.org/licenses/by-nc-nd/4.0/.
References
- Ilic M, Ilic I. Epidemiology of pancreatic cancer. World J Gastroenterol 2016;22:9694-705. [Crossref] [PubMed]
- Moore A, Donahue T. Pancreatic Cancer. JAMA 2019;322:1426. [Crossref] [PubMed]
- Ren B, Cui M, Yang G, et al. Tumor microenvironment participates in metastasis of pancreatic cancer. Mol Cancer 2018;17:108. [Crossref] [PubMed]
- Paget S. The distribution of secondary growths in cancer of the breast. 1889. Cancer Metastasis Rev 1989;8:98-101. [PubMed]
- Ewing J. Neoplastic Disease. A Treatise on Tumor. Am J Med Sci 1928;176:278. [Crossref]
- Fidler IJ, Nicolson GL. Organ selectivity for implantation survival and growth of B16 melanoma variant tumor lines. J Natl Cancer Inst 1976;57:1199-202. [Crossref] [PubMed]
- Peinado H, Zhang H, Matei IR, et al. Pre-metastatic niches: organ-specific homes for metastases. Nat Rev Cancer 2017;17:302-17. [Crossref] [PubMed]
- Pathan M, Keerthikumar S, Chisanga D, et al. A novel community driven software for functional enrichment analysis of extracellular vesicles data. J Extracell Vesicles 2017;6:1321455. [Crossref] [PubMed]
- STRING v11: protein-protein association networks with increased coverage, supporting functional discovery in genome-wide experimental datasets. Nucleic acids research 2019; [PubMed]
- Chandrashekar DS, Bashel B, Balasubramanya SAH, et al. UALCAN: A Portal for Facilitating Tumor Subgroup Gene Expression and Survival Analyses. Neoplasia 2017;19:649-58. [Crossref] [PubMed]
- Zefang T, Chenwei L, Boxi K, et al. GEPIA: a web server for cancer and normal gene expression profiling and interactive analyses. Nucleic Acids Res 2017;45:W98-W102. [Crossref] [PubMed]
- Li T, Fan J, Wang B, et al. TIMER: A Web Server for Comprehensive Analysis of Tumor-Infiltrating Immune Cells. Cancer Res 2017;77:e108-e110. [Crossref] [PubMed]
- Balachandran VP, Beatty GL, Dougan SK. Broadening the Impact of Immunotherapy to Pancreatic Cancer: Challenges and Opportunities. Gastroenterology 2019;156:2056-72. [Crossref] [PubMed]
- Houg DS, Bijlsma MF. The hepatic pre-metastatic niche in pancreatic ductal adenocarcinoma. Mol Cancer 2018;17:95. [Crossref] [PubMed]
- Mashouri L, Yousefi H, Aref AR, et al. Exosomes: composition, biogenesis, and mechanisms in cancer metastasis and drug resistance. Mol Cancer 2019;18:75. [Crossref] [PubMed]
- Chatterjee A, Gupta S. The multifaceted role of glutathione S-transferases in cancer. Cancer Lett 2018;433:33-42. [Crossref] [PubMed]
- Abel EV, Goto M, Magnuson B, et al. HNF1A is a novel oncogene that regulates human pancreatic cancer stem cell properties. Elife 2018;7:e33947. [Crossref] [PubMed]
- Eble JA, Niland S. The extracellular matrix in tumor progression and metastasis. Clin Exp Metastasis 2019;36:171-98. [Crossref] [PubMed]
- Sommariva M, Gagliano N. E-Cadherin in Pancreatic Ductal Adenocarcinoma: A Multifaceted Actor during EMT. Cells 2020;9:1040. [Crossref] [PubMed]
- Sheng W, Chen C, Dong M, et al. Calreticulin promotes EGF-induced EMT in pancreatic cancer cells via Integrin/EGFR-ERK/MAPK signaling pathway. Cell Death Dis 2017;8:e3147. [Crossref] [PubMed]
- Dong S, Huang F, Zhang H, et al. Overexpression of BUB1B, CCNA2, CDC20, and CDK1 in tumor tissues predicts poor survival in pancreatic ductal adenocarcinoma. Biosci Rep 2019;39:BSR20182306. [Crossref] [PubMed]
- Willder JM, Heng SJ, McCall P, et al. Androgen receptor phosphorylation at serine 515 by Cdk1 predicts biochemical relapse in prostate cancer patients. Br J Cancer 2013;108:139-48. [Crossref] [PubMed]
- Tsaur I, Makarević J, Hudak L, et al. The cdk1-cyclin B complex is involved in everolimus triggered resistance in the PC3 prostate cancer cell line. Cancer Lett 2011;313:84-90. [Crossref] [PubMed]
- Sung WW, Lin YM, Wu PR, et al. High nuclear/cytoplasmic ratio of Cdk1 expression predicts poor prognosis in colorectal cancer patients. BMC Cancer 2014;14:951. [Crossref] [PubMed]
- Banerjee SK, Weston AP, Zoubine MN, et al. Expression of cdc2 and cyclin B1 in Helicobacter pylori-associated gastric MALT and MALT lymphoma: relationship to cell death, proliferation, and transformation. Am J Pathol 2000;156:217-25. [Crossref] [PubMed]
- Li Y, Chen YL, Xie YT, et al. Association study of germline variants in CCNB1 and CDK1 with breast cancer susceptibility, progression, and survival among Chinese Han women. PLoS One 2013;8:e84489. [Crossref] [PubMed]
- Xi Q, Huang M, Wang Y, et al. The expression of CDK1 is associated with proliferation and can be a prognostic factor in epithelial ovarian cancer. Tumour Biol 2015;36:4939-48. [Crossref] [PubMed]
- Yang W, Cho H, Shin HY, et al. Accumulation of cytoplasmic Cdk1 is associated with cancer growth and survival rate in epithelial ovarian cancer. Oncotarget 2016;7:49481-97. [Crossref] [PubMed]
- Feng W, Cai D, Zhang B, et al. Combination of HDAC inhibitor TSA and silibinin induces cell cycle arrest and apoptosis by targeting survivin and cyclinB1/Cdk1 in pancreatic cancer cells. Biomed Pharmacother 2015;74:257-64. [Crossref] [PubMed]
- Costa-Cabral S, Brough R, Konde A, et al. CDK1 Is a Synthetic Lethal Target for KRAS Mutant Tumours. PLoS One 2016;11:e0149099. [Crossref] [PubMed]
- Zhang P, Chen L, Song Y, et al. Tetraiodothyroacetic acid and transthyretin silencing inhibit pro-metastatic effect of L-thyroxin in anoikis-resistant prostate cancer cells through regulation of MAPK/ERK pathway. Exp Cell Res 2016;347:350-9. [Crossref] [PubMed]
- Shimura T, Shibata M, Gonda K, et al. Serum transthyretin level is associated with prognosis of patients with gastric cancer. J Surg Res 2018;227:145-50. [Crossref] [PubMed]
- Lee CC, Ding X, Zhao T, et al. Transthyretin Stimulates Tumor Growth through Regulation of Tumor, Immune, and Endothelial Cells. J Immunol 2019;202:991-1002. [Crossref] [PubMed]
- Chen J, Chen LJ, Xia YL, et al. Identification and verification of transthyretin as a potential biomarker for pancreatic ductal adenocarcinoma. J Cancer Res Clin Oncol 2013;139:1117-27. [Crossref] [PubMed]
- Sancho P, Burgos-Ramos E, Tavera A, et al. MYC/PGC-1α Balance Determines the Metabolic Phenotype and Plasticity of Pancreatic Cancer Stem Cells. Cell Metab 2015;22:590-605. [Crossref] [PubMed]
Cite this article as: Chai C, Yang Z, Huang M, Xu J, Lu X. CDK1/TTR/MYC promote the formation of metastatic niches and affect the prognosis of pancreatic cancer by participating in immune CD4+ T cell infiltration as indicated by an integrated bioinformatics analysis. Dig Med Res 2020;3:40.